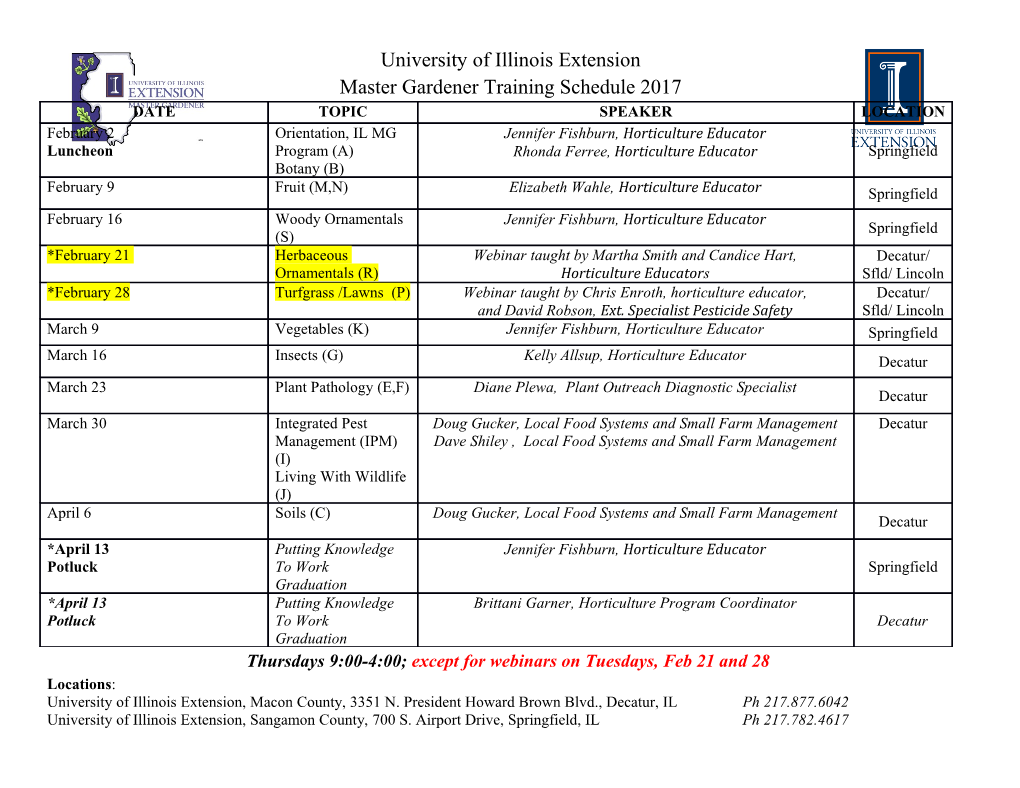
Lecture 10: Recurrent Neural Networks Fei-Fei Li, Ranjay Krishna, Danfei Xu Lecture 10 - 1 May 7, 2020 Administrative: Midterm - Midterm next Tue 5/12 take home - 1H 20 Mins (+20m buffer) within a 24 hour time period. - Will be released on Gradescope. - See Piazza for detailed information - Midterm review session: Fri 5/8 discussion section - Midterm covers material up to this lecture (Lecture 10) Fei-Fei Li, Ranjay Krishna, Danfei Xu Lecture 10 - 2 May 7, 2020 Administrative - Project proposal feedback has been released - Project milestone due Mon 5/18, see Piazza for requirements ** Need to have some baseline / initial results by then, so start implementing soon if you haven’t yet! - A3 will be released Wed 5/13, due Wed 5/27 Fei-Fei Li, Ranjay Krishna, Danfei Xu Lecture 10 - 3 May 7, 2020 Last Time: CNN Architectures GoogLeNet AlexNet Fei-Fei Li, Ranjay Krishna, Danfei Xu Lecture 10 - 4 May 7, 2020 Last Time: CNN Architectures ResNet SENet Fei-Fei Li, Ranjay Krishna, Danfei Xu Lecture 10 - 5 May 7, 2020 Comparing complexity... An Analysis of Deep Neural Network Models for Practical Applications, 2017. Figures copyright Alfredo Canziani, Adam Paszke, Eugenio Culurciello, 2017. Reproduced with permission. Fei-Fei Li, Ranjay Krishna, Danfei Xu Lecture 10 - 6 May 7, 2020 Efficient networks... MobileNets: Efficient Convolutional Neural Networks for Mobile Applications [Howard et al. 2017] - Depthwise separable convolutions replace BatchNorm standard convolutions by Pool BatchNorm factorizing them into a C2HW Pointwise Conv (1x1, C->C) convolutions depthwise convolution and a Pool 1x1 convolution BatchNorm 9C2HW Conv (3x3, C->C) - Much more efficient, with Pool little loss in accuracy Stanford network Conv (3x3, C->C, Depthwise 2 9CHW - Follow-up MobileNetV2 work Total compute:9C HW groups=C) convolutions in 2018 (Sandler et al.) MobileNets - ShuffleNet: Zhang et al, Total compute:9CHW + C2HW CVPR 2018 Fei-Fei Li, Ranjay Krishna, Danfei Xu Lecture 10 - May 7, 2020 Meta-learning: Learning to learn network architectures... Neural Architecture Search with Reinforcement Learning (NAS) [Zoph et al. 2016] - “Controller” network that learns to design a good network architecture (output a string corresponding to network design) - Iterate: 1) Sample an architecture from search space 2) Train the architecture to get a “reward” R corresponding to accuracy 3) Compute gradient of sample probability, and scale by R to perform controller parameter update (i.e. increase likelihood of good architecture being sampled, decrease likelihood of bad architecture) Fei-Fei Li, Ranjay Krishna, Danfei Xu Lecture 10 - 8 May 7, 2020 Meta-learning: Learning to learn network architectures... Learning Transferable Architectures for Scalable Image Recognition [Zoph et al. 2017] - Applying neural architecture search (NAS) to a large dataset like ImageNet is expensive - Design a search space of building blocks (“cells”) that can be flexibly stacked - NASNet: Use NAS to find best cell structure on smaller CIFAR-10 dataset, then transfer architecture to ImageNet - Many follow-up works in this space e.g. AmoebaNet (Real et al. 2019) and ENAS (Pham, Guan et al. 2018) Fei-Fei Li, Ranjay Krishna, Danfei Xu Lecture 10 - 9 May 7, 2020 Today: Recurrent Neural Networks Fei-Fei Li, Ranjay Krishna, Danfei Xu Lecture 10 - 10 May 7, 2020 “Vanilla” Neural Network Vanilla Neural Networks Fei-Fei Li, Ranjay Krishna, Danfei Xu Lecture 10 - 11 May 7, 2020 Recurrent Neural Networks: Process Sequences e.g. Image Captioning image -> sequence of words Fei-Fei Li, Ranjay Krishna, Danfei Xu Lecture 10 - 12 May 7, 2020 Recurrent Neural Networks: Process Sequences e.g. action prediction sequence of video frames -> action class Fei-Fei Li, Ranjay Krishna, Danfei Xu Lecture 10 - 13 May 7, 2020 Recurrent Neural Networks: Process Sequences E.g. Video Captioning Sequence of video frames -> caption Fei-Fei Li, Ranjay Krishna, Danfei Xu Lecture 10 - 14 May 7, 2020 Recurrent Neural Networks: Process Sequences e.g. Video classification on frame level Fei-Fei Li, Ranjay Krishna, Danfei Xu Lecture 10 - 15 May 7, 2020 Sequential Processing of Non-Sequence Data Classify images by taking a series of “glimpses” Ba, Mnih, and Kavukcuoglu, “Multiple Object Recognition with Visual Attention”, ICLR 2015. Gregor et al, “DRAW: A Recurrent Neural Network For Image Generation”, ICML 2015 Figure copyright Karol Gregor, Ivo Danihelka, Alex Graves, Danilo Jimenez Rezende, and Daan Wierstra, 2015. Reproduced with permission. Fei-Fei Li, Ranjay Krishna, Danfei Xu Lecture 10 - 16 May 7, 2020 Sequential Processing of Non-Sequence Data Generate images one piece at a time! Gregor et al, “DRAW: A Recurrent Neural Network For Image Generation”, ICML 2015 Figure copyright Karol Gregor, Ivo Danihelka, Alex Graves, Danilo Jimenez Rezende, and Daan Wierstra, 2015. Reproduced with permission. Fei-Fei Li, Ranjay Krishna, Danfei Xu Lecture 10 - 17 May 7, 2020 Recurrent Neural Network y RNN x Fei-Fei Li, Ranjay Krishna, Danfei Xu Lecture 10 - 18 May 7, 2020 Recurrent Neural Network y Key idea: RNNs have an “internal state” that is updated as a sequence is RNN processed x Fei-Fei Li, Ranjay Krishna, Danfei Xu Lecture 10 - 19 May 7, 2020 Recurrent Neural Network y y y 1 2 3 yt RNN RNN RNN ... RNN x x x 1 2 3 xt Fei-Fei Li, Ranjay Krishna, Danfei Xu Lecture 10 - 20 May 7, 2020 Recurrent Neural Network We can process a sequence of vectors x by applying a recurrence formula at every time step: y RNN new state old state input vector at some time step some function x with parameters W Fei-Fei Li, Ranjay Krishna, Danfei Xu Lecture 10 - 21 May 7, 2020 Recurrent Neural Network y y y 1 2 3 yt h0 h h h RNN 1 RNN 2 RNN 3 ... RNN x x x 1 2 3 xt Fei-Fei Li, Ranjay Krishna, Danfei Xu Lecture 10 - 22 May 7, 2020 Recurrent Neural Network We can process a sequence of vectors x by applying a recurrence formula at every time step: y RNN Notice: the same function and the same set x of parameters are used at every time step. Fei-Fei Li, Ranjay Krishna, Danfei Xu Lecture 10 - 23 May 7, 2020 (Simple) Recurrent Neural Network The state consists of a single “hidden” vector h: y RNN x Sometimes called a “Vanilla RNN” or an “Elman RNN” after Prof. Jeffrey Elman Fei-Fei Li, Ranjay Krishna, Danfei Xu Lecture 10 - 24 May 7, 2020 RNN: Computational Graph h h 0 fW 1 x1 Fei-Fei Li, Ranjay Krishna, Danfei Xu Lecture 10 - 25 May 7, 2020 RNN: Computational Graph h h h 0 fW 1 fW 2 x1 x2 Fei-Fei Li, Ranjay Krishna, Danfei Xu Lecture 10 - 26 May 7, 2020 RNN: Computational Graph h f h f h f h h 0 W 1 W 2 W 3 … T x1 x2 x3 Fei-Fei Li, Ranjay Krishna, Danfei Xu Lecture 10 - 27 May 7, 2020 RNN: Computational Graph Re-use the same weight matrix at every time-step h f h f h f h h 0 W 1 W 2 W 3 … T x x x W 1 2 3 Fei-Fei Li, Ranjay Krishna, Danfei Xu Lecture 10 - 28 May 7, 2020 RNN: Computational Graph: Many to Many y y y y1 2 3 T h f h f h f h h 0 W 1 W 2 W 3 … T x x x W 1 2 3 Fei-Fei Li, Ranjay Krishna, Danfei Xu Lecture 10 - 29 May 7, 2020 RNN: Computational Graph: Many to Many y L y L y L y1 L1 2 2 3 3 T T h f h f h f h h 0 W 1 W 2 W 3 … T x x x W 1 2 3 Fei-Fei Li, Ranjay Krishna, Danfei Xu Lecture 10 - 30 May 7, 2020 L RNN: Computational Graph: Many to Many y L y L y L y1 L1 2 2 3 3 T T h f h f h f h h 0 W 1 W 2 W 3 … T x x x W 1 2 3 Fei-Fei Li, Ranjay Krishna, Danfei Xu Lecture 10 - 31 May 7, 2020 RNN: Computational Graph: Many to One y h f h f h f h h 0 W 1 W 2 W 3 … T x x x W 1 2 3 Fei-Fei Li, Ranjay Krishna, Danfei Xu Lecture 10 - 32 May 7, 2020 RNN: Computational Graph: Many to One y h f h f h f h h 0 W 1 W 2 W 3 … T x x x W 1 2 3 Fei-Fei Li, Ranjay Krishna, Danfei Xu Lecture 10 - 33 May 7, 2020 RNN: Computational Graph: One to Many y y y y1 2 3 T h f h f h f h h 0 W 1 W 2 W 3 … T x W Fei-Fei Li, Ranjay Krishna, Danfei Xu Lecture 10 - 34 May 7, 2020 RNN: Computational Graph: One to Many y y y y1 2 3 T h f h f h f h h 0 W 1 W 2 W 3 … T x ? ? W ? Fei-Fei Li, Ranjay Krishna, Danfei Xu Lecture 10 - 35 May 7, 2020 RNN: Computational Graph: One to Many y y y y1 2 3 T h f h f h f h h 0 W 1 W 2 W 3 … T x 0 0 W 0 Fei-Fei Li, Ranjay Krishna, Danfei Xu Lecture 10 - 36 May 7, 2020 RNN: Computational Graph: One to Many y y y y1 2 3 T h f h f h f h h 0 W 1 W 2 W 3 … T x y y yT-1 W 1 2 Fei-Fei Li, Ranjay Krishna, Danfei Xu Lecture 10 - 37 May 7, 2020 Sequence to Sequence: Many-to-one + one-to-many Many to one: Encode input sequence in a single vector h h h h … h 0 fW 1 fW 2 fW 3 T x1 x2 x3 W1 Sutskever et al, “Sequence to Sequence Learning with Neural Networks”, NIPS 2014 Fei-Fei Li, Ranjay Krishna, Danfei Xu Lecture 10 - 38 May 7, 2020 Sequence to Sequence: Many-to-one + one-to-many One to many: Produce output sequence from single input vector Many to one: Encode input sequence in a single vector y1 y2 h h h h … h 0 f 1 f 2 f 3 T h h … W W W fW 1 fW 2 fW x1 x2 x3 W 1 W2 Sutskever et al, “Sequence to Sequence Learning with Neural Networks”, NIPS 2014 Fei-Fei Li, Ranjay Krishna, Danfei Xu Lecture 10 - 39 May 7, 2020 Example: Character-level Language Model Vocabulary: [h,e,l,o] Example training sequence: “hello” Fei-Fei Li, Ranjay Krishna, Danfei Xu Lecture 10 - 40 May 7, 2020 Example: Character-level Language Model Vocabulary: [h,e,l,o] Example training sequence: “hello” Fei-Fei Li, Ranjay Krishna, Danfei Xu Lecture 10 - 41 May 7, 2020 Example: Character-level Language Model Vocabulary: [h,e,l,o] Example training sequence: “hello” Fei-Fei Li, Ranjay Krishna, Danfei Xu Lecture 10 - 42 May 7, 2020 “e” “l” “l” “o” Example: Sample .03 .25 .11 .11 Character-level .84 .20 .17 .02 Softmax
Details
-
File Typepdf
-
Upload Time-
-
Content LanguagesEnglish
-
Upload UserAnonymous/Not logged-in
-
File Pages128 Page
-
File Size-