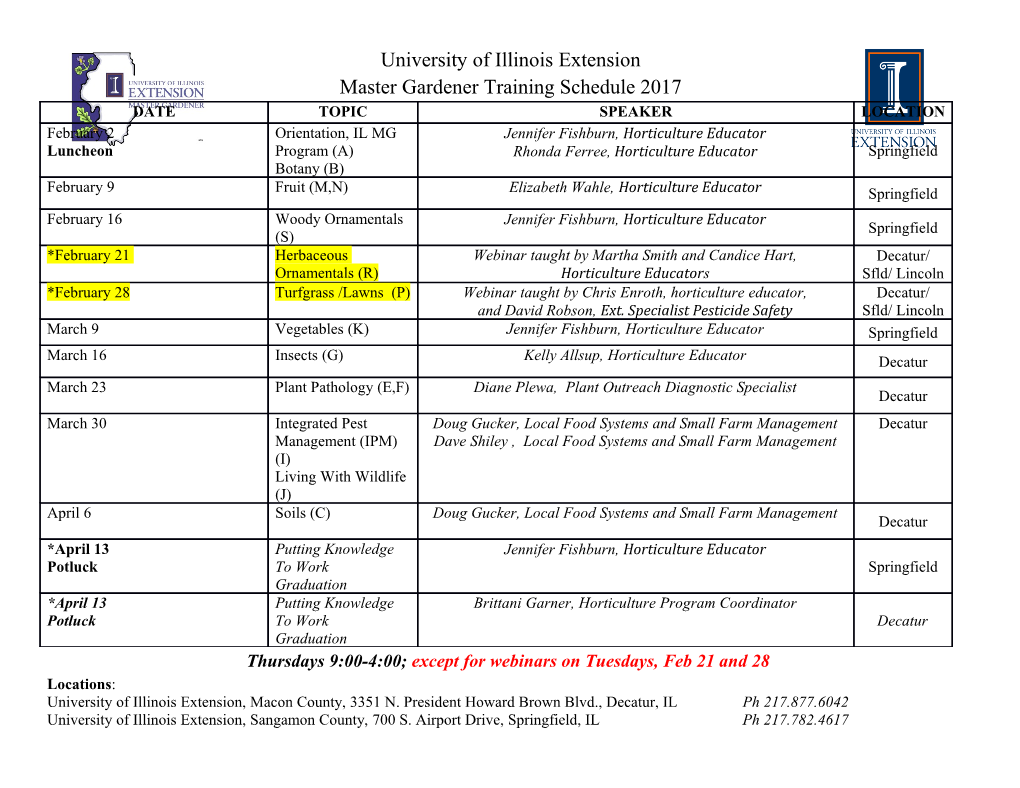
Choi and Nam BMC Bioinformatics 2018, 19(Suppl 1):41 DOI 10.1186/s12859-018-2013-9 RESEARCH Open Access TERIUS: accurate prediction of lncRNA via high-throughput sequencing data representing RNA-binding protein association Seo-Won Choi1 and Jin-Wu Nam1,2* From Proceedings of the 28th International Conference on Genome Informatics: bioinformatics Seoul, Korea. 31 October - 3 November 2017 Abstract Background: LncRNAs are long regulatory non-coding RNAs, some of which are arguably predicted to have coding potential. Despite coding potential classifiers that utilize ribosome profiling data successfully detected actively translated regions, they are less sensitive to lncRNAs. Furthermore, lncRNA annotation can be susceptible to false positives obtained from 3′ untranslated region (UTR) fragments of mRNAs. Results: To lower these limitations in lncRNA annotation, we present a novel tool TERIUS that provides a two-step filtration process to distinguish between bona fide and false lncRNAs. The first step successfully separates lncRNAs from protein-coding genes showing enhanced sensitivity compared to other methods. To eliminate 3’UTR fragments, the second step takes advantage of the 3’UTR-specific association with regulator of nonsense transcripts 1 (UPF1), leading to refined lncRNA annotation. Importantly, TERIUS enabled the detection of misclassified transcripts in published lncRNA annotations. Conclusions: TERIUS is a robust method for lncRNA annotation, which provides an additional filtration step for 3’UTR fragments. TERIUS was able to successfully re-classify GENCODE and miTranscriptome lncRNA annotations. We believe that TERIUS can benefit construction of extensive and accurate non-coding transcriptome maps in many genomes. Keywords: LncRNA, LncRNA annotation, RNA binding protein association Background of lncRNAs across different species and cell lines [5–7]. Long non-coding RNAs (lncRNAs) are a group of regu- Meanwhile, other work has led to different conclusions, latory non-coding RNAs (ncRNAs) that are involved in including that lncRNAs are deprived of functional open diverse biological processes [1]. Despite developments in reading frames (ORFs) [8, 9] or that some lncRNAs are research, lncRNA is still poorly defined and therefore actively translated [6, 10, 11]. Other research reports suffers from erroneous annotation [2–4]. For one, the that some lncRNAs are capable of coding short func- coding potential of lncRNA has long been debated, tional peptides in mice [12, 13], and that lncRNAs may regardless of the fact that its name harbors the term be bifunctional, with coding and non-coding isoforms “non-coding.” Several studies have reported unexplained reacting to cell conditions [14, 15]. Unfortunately, the associations between ribosomes and varying proportions extent to which these ribosome-associated lncRNAs are translated remains unknown. * Correspondence: [email protected] To resolve this question, pioneers in the lncRNA field 1Department of Life Science, College of Natural Sciences, Hanyang University, developed several algorithms to identify translated tran- Seoul 04763, Republic of Korea 2Research Institute for Convergence of Basic Sciences, Hanyang University, scripts by sensing their intrinsic characters, such as the Seoul 04763, Republic of Korea ORF length [16–18], sequence similarity to known © The Author(s). 2018 Open Access This article is distributed under the terms of the Creative Commons Attribution 4.0 International License (http://creativecommons.org/licenses/by/4.0/), which permits unrestricted use, distribution, and reproduction in any medium, provided you give appropriate credit to the original author(s) and the source, provide a link to the Creative Commons license, and indicate if changes were made. The Creative Commons Public Domain Dedication waiver (http://creativecommons.org/publicdomain/zero/1.0/) applies to the data made available in this article, unless otherwise stated. Choi and Nam BMC Bioinformatics 2018, 19(Suppl 1):41 Page 24 of 104 proteins [19], conservation [16, 20], and codon usage or transcripts. The second step, involves calculation of the UPF1 kmer bias [16–18, 21]. More recently, following the foot- association score (UAS) that detects invalid lncRNAs. steps of Ingolia et al. and Guttman et al. [9, 22], several studies have explored coding potential prediction within Methods the context of in vivo translation using ribosome profil- Processing of high-throughput sequence data ing data. Ingolia and his colleagues defined translation All Ribo-seq and RNA-seq sequence data used in this efficiency (TE) as the approximation of effective ribo- study were downloaded from the NCBI gene expression some engagement to RNAs, and Guttman and his group omnibus (GEO) dataset [26] and aligned to reference ge- conceived a program, the ribosome release score (RRS), nomes (hg19 for human and mm9 for mouse) using focused on ribosome disengagement at the start of TopHat version 2.0.6 [27] with alignment options -g 1 3’UTR region. The translated ORF classifier (TOC) de- –b2-N 0 –b2-L 20, intron options -i 61 -I 265006 for veloped by Chew et al. also employed a similar feature the human sequence, and -i 52 -I 240764 for the mouse [8]. Bazzini et al. devised the ORFscore that predicts the sequence (Additional file 1: Table S1). coding potential of ORFs by quantifying the biased dis- Crosslinking immunoprecipitation sequencing (CLIP- tribution of ribosome reads toward the first frame by seq) BED files were downloaded from the GEO dataset, testing observed ribosome read distributions under the converted to BAM files using BEDtools version 2.17.0 null hypothesis of Chi-squared test [23]. Subsequently, [28], and then modified with an in-house Python script Calveilo et al. devised a more sophisticated program, to add reads according to BED signal intensity. Three RiboTaper, coupling ribosome periodicity with Fourier mouse CLIP-seq replicates were merged and the mean transformation strengthened by a multitaper approach values were obtained. All gene expression or association [24]. Rather than imposing a hypothetical uniform distri- levels were calculated using an in-house Python script. bution like in the ORFscore, RiboTaper compares the CAGE-seq and poly(A)-position profiling by sequen- spectra derived from ribosome protected fragment se- cing (3P–seq) BED files were downloaded from the quencing (Ribo-seq) to those from high-throughput FANTOM 5 project [29] and from NCBI GEO datasets RNA sequencing (RNA-seq) to ensure capture of signifi- (Additional file 1: Table S1). cant peaks of frequency representing periodicity. Despite that current strategies can be effective for the detection Training and test datasets of conserved, highly expressed, classic protein-coding To define the training and test gene annotations for genes, they may not be appropriate for the identification TERIUS, the RefSeq protein-coding gene annotation of young, less productive genes with short ORFs that are (version 2013.09.09 for human and 2014.11.23 for the center of the ongoing debate. mouse) [30], GENCODE annotation version v19 [2] for Other than the ambiguity regarding the translated ncRNA genes, and Vertebrate Genome Annotation lncRNA subpopulation, the non-coding group also suf- (VEGA) lncRNA annotation (version 54 for human and fers from debatable annotations. As novel transcripts are version 68 for mouse) [31] were downloaded. Annota- assembled based on RNA-seq signals, where only the tions based on mm10 genome assembly were converted coding potential is assessed before they are annotated as to the mm9 assembly using the liftOver tool [32]. Col- lncRNAs, the integrity of lncRNA annotation is barely lected annotations were then subjected to downstream protected from the non-coding fragments of other genes. processes for RPS followed by UAS. Above all, the 3’UTR region of protein-coding genes is the For the RPS, the protein-coding gene isoforms with leading candidate for such fragments as it tends to show the longest coding sequence (CDS) were selected. To weak, long, and fragmented RNA-seq signals that stretch generate positive (non-coding) data, classical non-coding downstream. Nonetheless, no appropriate solution has RNAs (rRNA, snoRNA, snRNA, miRNA, and so on) been suggested for the detection of false annotations. were collected from GENCODE v19 annotations. Although the use of cap analysis of gene expression Among collected ncRNA annotation, those with protein- (CAGE) and polyadenylation tags for the determination of coding potential was filtered out using CPC [19] and transcript boundaries has been quite effective [5, 25], the CPAT [18]. Default cutoffs and logistic model provided required data are not available for most model organisms by each program were used. Remaining ncRNAs were and cell types. again searched against UniProt database [33] to elimin- To address these problems, we introduce the Translation- ate any genes with reported protein-coding entries. Then dependent Ensemble classifier with RIbosome and UPF1 as- for each coding and non-coding gene, Ribo-seq signals sociation Score (TERIUS) that is robust and can successfully for the sub-codon positions were counted and those that refine lncRNA annotations using a two-step paradigm. The lacked signal in any of the three positions were first step, involves calculation of the ribosome periodicity discarded, leaving 7710 mRNAs and 92 ncRNAs. To score (RPS) and is responsible for separating coding account for the size imbalance between ncRNAs and Choi and Nam
Details
-
File Typepdf
-
Upload Time-
-
Content LanguagesEnglish
-
Upload UserAnonymous/Not logged-in
-
File Pages9 Page
-
File Size-