Link Prediction in Networks with Nodes Attributes by Similarity
Total Page:16
File Type:pdf, Size:1020Kb
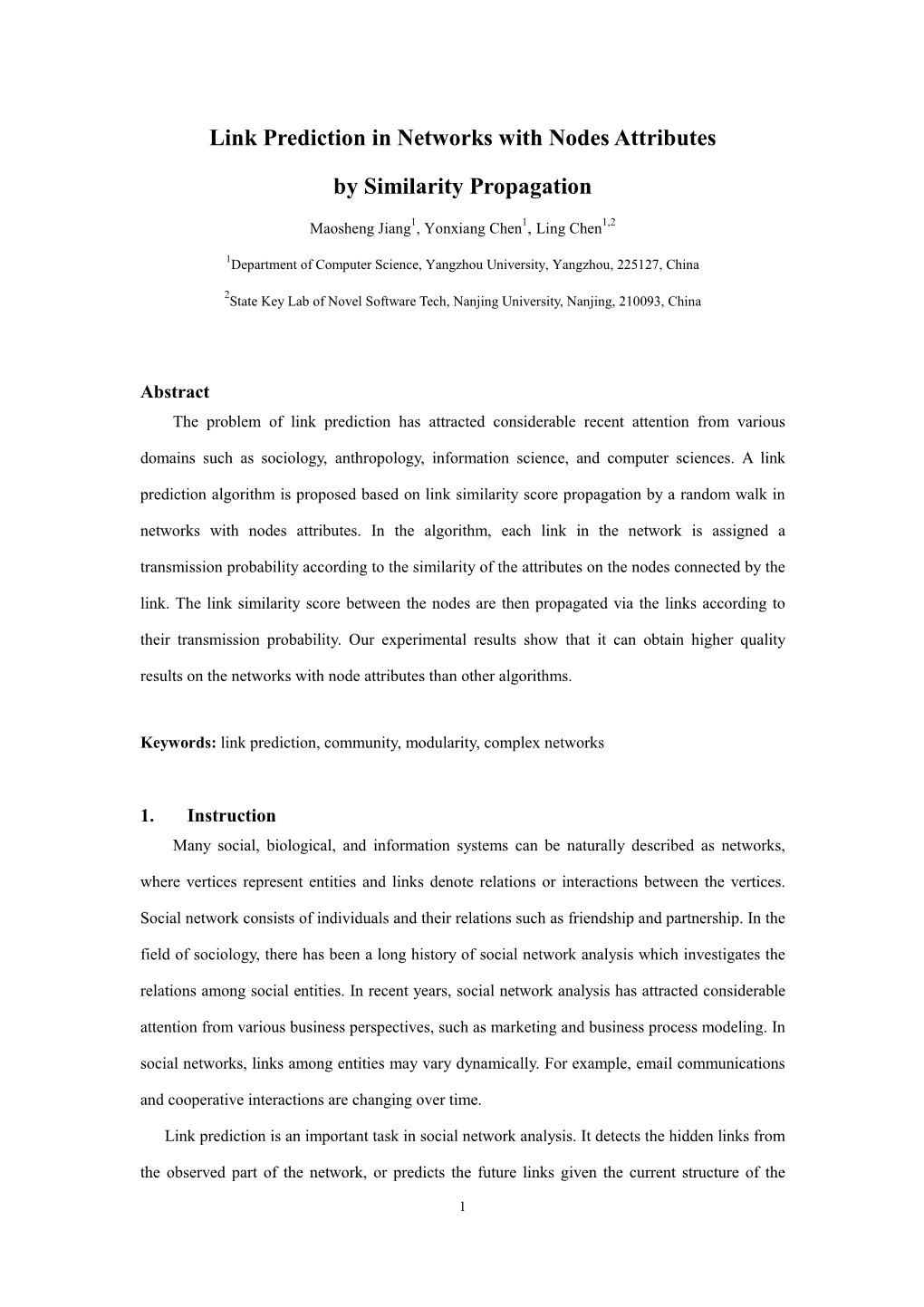
Load more
Recommended publications
-
Biased Edge Dropout for Enhancing Fairness in Graph Representation
PREPRINT SUBMITTED TO A JOURNAL. 1 Biased Edge Dropout for Enhancing Fairness in Graph Representation Learning Indro Spinelli, Graduate Student Member, IEEE, Simone Scardapane, Amir Hussain, Senior Member, IEEE and Aurelio Uncini, Member, IEEE Abstract—Graph representation learning has become a ubiqui- I. INTRODUCTION tous component in many scenarios, ranging from social network analysis to energy forecasting in smart grids. In several applica- RAPH structured data, ranging from friendships on tions, ensuring the fairness of the node (or graph) representations G social networks to physical links in energy grids, powers with respect to some protected attributes is crucial for their many algorithms governing our digital life. Social networks correct deployment. Yet, fairness in graph deep learning remains under-explored, with few solutions available. In particular, the topologies define the stream of information we will receive, tendency of similar nodes to cluster on several real-world often influencing our opinion [1][2][3][4]. Bad actors, some- graphs (i.e., homophily) can dramatically worsen the fairness times, define these topologies ad-hoc to spread false informa- of these procedures. In this paper, we propose a biased edge tion [5]. Similarly, recommender systems [6] suggest products dropout algorithm (FairDrop) to counter-act homophily and tailored to our own experiences and history of purchases. improve fairness in graph representation learning. FairDrop can be plugged in easily on many existing algorithms, is efficient, However, pursuing the highest accuracy as the only metric of adaptable, and can be combined with other fairness-inducing interest has let many of these algorithms discriminate against solutions. After describing the general algorithm, we demonstrate minorities in the past [7][8][9], despite the law prohibiting its application on two benchmark tasks, specifically, as a random unfair treatment based on sensitive traits such as race, religion, walk model for producing node embeddings, and to a graph and gender. -
Combining Collective Classification and Link Prediction
Combining Collective Classification and Link Prediction Mustafa Bilgic, Galileo Mark Namata, Lise Getoor Dept. of Computer Science Univ. of Maryland College Park, MD 20742 {mbilgic, namatag, getoor}@cs.umd.edu Abstract however, this is rarely the case. Real world collections usu- ally have a number of missing and incorrect labels and links. The problems of object classification (labeling the nodes Other approaches construct a complex joint probabilistic of a graph) and link prediction (predicting the links in model, capable of handling missing values, but in which a graph) have been largely studied independently. Com- inference is often intractable. monly, object classification is performed assuming a com- In this paper, we take the middle road. We propose a plete set of known links and link prediction is done assum- simple yet general approach for combining object classifi- ing a fully observed set of node attributes. In most real cation and link prediction. We propose an algorithm called world domains, however,attributes and links are often miss- Iterative Collective Classification and Link Prediction (IC- ing or incorrect. Object classification is not provided with CLP) that integrates collective object classification and link all the links relevant to correct classification and link pre- prediction by effectively passing up-to-date information be- diction is not provided all the labels needed for accurate tween the algorithms. We experimentally show on many link prediction. In this paper, we propose an approach that different network types that applying ICCLP improves per- addresses these two problems by interleaving object clas- formance over running collective classification and link pre- sification and link prediction in a collective algorithm. -
Finding Experts by Link Prediction in Co-Authorship Networks
Finding Experts by Link Prediction in Co-authorship Networks Milen Pavlov1,2,RyutaroIchise2 1 University of Waterloo, Waterloo ON N2L 3G1, Canada 2 National Institute of Informatics, Tokyo 101-8430, Japan Abstract. Research collaborations are always encouraged, as they of- ten yield good results. However, the researcher network contains massive amounts of experts in various disciplines and it is difficult for the indi- vidual researcher to decide which experts will match his own expertise best. As a result, collaboration outcomes are often uncertain and research teams are poorly organized. We propose a method for building link pre- dictors in networks, where nodes can represent researchers and links - collaborations. In this case, predictors might offer good suggestions for future collaborations. We test our method on a researcher co-authorship network and obtain link predictors of encouraging accuracy. This leads us to believe our method could be useful in building and maintaining strong research teams. It could also help with choosing vocabulary for expert de- scription, since link predictors contain implicit information about which structural attributes of the network are important with respect to the link prediction problem. 1 Introduction Collaborations between researchers often have a synergistic effect. The combined expertise of a group of researchers can often yield results far surpassing the sum of the individual researchers’ capabilities. However, creating and organizing such research teams is not a straightforward task. The individual researcher often has limited awareness of the existence of other researchers with which collaborations might prove fruitful. Further- more, even in the presence of such awareness, it is difficult to predict in advance which potential collaborations should be pursued. -
Learning to Make Predictions on Graphs with Autoencoders
Learning to Make Predictions on Graphs with Autoencoders Phi Vu Tran Strategic Innovation Group Booz j Allen j Hamilton San Diego, CA USA [email protected] Abstract—We examine two fundamental tasks associated networks [38], communication networks [11], cybersecurity with graph representation learning: link prediction and semi- [6], recommender systems [16], and knowledge bases such as supervised node classification. We present a novel autoencoder DBpedia and Wikidata [35]. architecture capable of learning a joint representation of both local graph structure and available node features for the multi- There are a number of technical challenges associated with task learning of link prediction and node classification. Our learning to make meaningful predictions on complex graphs: autoencoder architecture is efficiently trained end-to-end in a single learning stage to simultaneously perform link prediction • Extreme class imbalance: in link prediction, the number and node classification, whereas previous related methods re- of known present (positive) edges is often significantly quire multiple training steps that are difficult to optimize. We less than the number of known absent (negative) edges, provide a comprehensive empirical evaluation of our models making it difficult to reliably learn from rare examples; on nine benchmark graph-structured datasets and demonstrate • Learn from complex graph structures: edges may be significant improvement over related methods for graph rep- resentation learning. Reference code and data are available at directed or undirected, weighted or unweighted, highly https://github.com/vuptran/graph-representation-learning. sparse in occurrence, and/or consisting of multiple types. Index Terms—network embedding, link prediction, semi- A useful model should be versatile to address a variety supervised learning, multi-task learning of graph types, including bipartite graphs; • Incorporate side information: nodes (and maybe edges) I. -
Predicting Disease Related Microrna Based on Similarity and Topology
cells Article Predicting Disease Related microRNA Based on Similarity and Topology Zhihua Chen 1,†, Xinke Wang 2,†, Peng Gao 2,†, Hongju Liu 3,† and Bosheng Song 4,* 1 Institute of Computing Science and Technology, Guangzhou University, Guangzhou 510006, China; [email protected] 2 School of Artificial Intelligence and Automation, Huazhong University of Science and Technology, Wuhan 430074, China; [email protected] (X.W.); [email protected] (P.G.) 3 College of Information Technology and Computer Science, University of the Cordilleras, Baguio 2600, Philippines; [email protected] 4 School of Information Science and Engineering, Hunan University, Changsha 410082, China * Correspondence: [email protected]; Tel.: +86-731-88821907 † All authors contributed equally to this work. Received: 4 July 2019; Accepted: 5 November 2019; Published: 7 November 2019 Abstract: It is known that many diseases are caused by mutations or abnormalities in microRNA (miRNA). The usual method to predict miRNA disease relationships is to build a high-quality similarity network of diseases and miRNAs. All unobserved associations are ranked by their similarity scores, such that a higher score indicates a greater probability of a potential connection. However, this approach does not utilize information within the network. Therefore, in this study, we propose a machine learning method, called STIM, which uses network topology information to predict disease–miRNA associations. In contrast to the conventional approach, STIM constructs features according to information on similarity and topology in networks and then uses a machine learning model to predict potential associations. To verify the reliability and accuracy of our method, we compared STIM to other classical algorithms. -
Representation Learning on Graphs
Representation learning on graphs A/Prof Truyen Tran [email protected] @truyenoz Deakin University truyentran.github.io letdataspeak.blogspot.com goo.gl/3jJ1O0 HCMC, May 2019 11/05/2019 1 Graph representation Graph reasoning Why bother? Graph generation Embedding Graph dynamics Message passing 11/05/2019 2 Why learning of graph representation? Graphs are pervasive in many scientific disciplines. The sub-area of graph representation has reached a certain maturity, with multiple reviews, workshops and papers at top AI/ML venues. Deep learning needs to move beyond vector, fixed-size data. Learning representation as a powerful way to discover hidden patterns making learning, inference and planning easier. 11/05/2019 3 System medicine 11/05/2019 https://www.frontiersin.org/articles/10.3389/fphys.2015.00225/full 4 Biology & pharmacy Traditional techniques: Graph kernels (ML) Molecular fingerprints (Chemistry) Modern techniques Molecule as graph: atoms as nodes, chemical bonds as edges #REF: Penmatsa, Aravind, Kevin H. Wang, and Eric Gouaux. "X- ray structure of dopamine transporter elucidates antidepressant mechanism." Nature 503.7474 (2013): 85-90. 11/05/2019 5 Chemistry DFT = Density Functional Theory Gilmer, Justin, et al. "Neural message passing for quantum chemistry." arXiv preprint arXiv:1704.01212 (2017). • Molecular properties • Chemical-chemical interaction • Chemical reaction • Synthesis planning 11/05/2019 6 Materials science • Crystal properties • Exploring/generating solid structures • Inverse design Xie, Tian, and Jeffrey -
Khanamk2021m-1A.Pdf (2.656Mb)
Lakehead University Knowledge Commons,http://knowledgecommons.lakeheadu.ca Electronic Theses and Dissertations Electronic Theses and Dissertations from 2009 2021 Using homophily to analyze and develop link prediction models with deep learning framework Khanam, Kazi Zainab https://knowledgecommons.lakeheadu.ca/handle/2453/4829 Downloaded from Lakehead University, KnowledgeCommons Using Homophily to Analyze and Develop Link Prediction Models with Deep Learning Framework by Kazi Zainab Khanam A thesis submitted in partial fulfillment of the requirements for the degree of Master of Science in Computer Science in the Faculty of Science and Environmental Studies of Lakehead University, Thunder Bay Committee in charge: Dr. Vijay Mago (Principal Supervisor) Dr. Rajesh Sharma (External Examiner) Dr. Yimin Yang (Internal Examiner) Winter 2021 The thesis of Kazi Zainab Khanam, titled Using Homophily to Analyze and Develop Link Prediction Models with Deep Learning Framework, is approved: Chair Date Date Date Lakehead University, Thunder Bay Using Homophily to Analyze and Develop Link Prediction Models with Deep Learning Framework Copyright 2021 by Kazi Zainab Khanam 1 Abstract USING HOMOPHILY TO ANALYZE AND DEVELOP LINK PREDICTION MODELS WITH DEEP LEARNING FRAMEWORK Twitter is a prominent social networking platform where users’ short messages or “tweets” are often used for analysis. However, there has not been much attention paid to mining the medical professions, such as detecting users’ occupations from their biographical content. Mining such information can be useful to build recommender systems for cost-effective ad- vertisements. Conventional classifiers can be used to predict medical occupations, but they tend to perform poorly as there are a variety of occupations. As a result, the main focus of the research is to use various deep learning techniques to examine the textual properties of Twitter users’ biographic contents, network properties, and the impact of homophily of Twitter users employed in medical professional fields. -
Neural Variational Inference for Embedding Knowledge Graphs
University College London MSc Data Science and Machine Learning Neural Variational Inference For Embedding Knowledge Graphs Author: Supervisor: Alexander Cowen-Rivers Prof. Sebastian Riedel Disclaimer This report is submitted as part requirement for the MSc Degree in Data Science and Machine Learning at University College London. It is substantially the result of my own work except where explicitly indicated in the text. The report may be freely copied and distributed provided the source is explicitly acknowledged.The report may be freely copied and distributed provided the source is explicitly acknowledged. Machine Reading Group October 23, 2018 i \Probability is expectation founded upon partial knowledge. A perfect acquaintance with all the circumstances affecting the occurrence of an event would change expectation into certainty, and leave nether room nor demand for a theory of probabilities." George Boole ii Acknowledgements I would like to thank my supervisors Prof Sebastian Riedel, as well as the other Machine Reading group members, particularly Dr Minervini, who helped through comments and discussions during the writing of this thesis. I would also like to thank my father who nurtured my passion for mathematics. Lastly, I would like to thank Thomas Kipf from the University of Amsterdam, who cleared up some queries I had regarding some of his recent work. iii Abstract Alexander Cowen-Rivers Neural Variational Inference For Embedding Knowledge Graphs Statistical relational learning investigates the development of tools to study graph-structured data. In this thesis, we provide an introduction on how models of the world are learnt using knowledge graphs of known facts of information, later applied to infer new facts about the world. -
A Scalable and Distributed Actor-Based Version of the Node2vec Algorithm
Workshop "From Objects to Agents" (WOA 2019) A Scalable and Distributed Actor-Based Version of the Node2Vec Algorithm Gianfranco Lombardo Agostino Poggi Department of Engineering and Architecture Department of Engineering and Architecture University of Parma University of Parma Parma, Italy Parma, Italy [email protected] [email protected] Abstract—The analysis of systems that can be modeled as attributed networks: an interaction network and a friendship networks of interacting entities is becoming often more important network. In [3] the authors analyze the same community in in different research fields. The application of machine learning order to extract the giant component without using topology algorithms, like prediction tasks over nodes and edges, requires a manually feature extraction or a learning task to extract them information with a bio-inspired algorithm. In [4] the authors automatically (embedding techniques). Several approaches have uses a temporal network to model the USA financial market in been proposed in the last years and the most promising one order to discover correlations among the dynamics of stocks’ is represented by the Node2Vec algorithm. However, common cluster and to predict economic crises. In [5] the authors limitations of graph embedding techniques are related to memory modeled the interaction between proteins as a network, with requirements and to the time complexity. In this paper, we propose a scalable and distributed version of this algorithm called the aim of automatic predicting a correct label for each protein ActorNode2vec, developed on an actor-based architecture that describing its functionalities. In light of this, this formulation allows to overcome these kind of constraints. -
Application of Machine Learning to Link Prediction
Application of Machine Learning to Link Prediction Kyle Julian (kjulian3), Wayne Lu (waynelu) December 16, 2016 1 Introduction Real-world networks evolve over time as new nodes and links are added. Link prediction algorithms use historical data in order to predict the appearance of a new links in the network or to identify links which may exist but are not represented in the data. The application of link prediction is most commonly seen in recommendation engines, such as new connections on social networks or related products on shopping sites. Traditional approaches involve the calculation of a heuristic similarity score for a pair of nodes, such as the number of common neighbors or the shortest path length connecting the nodes, where pairs of nodes with the highest similarity scores are considered the most likely edges. In this project, we will apply supervised learning algorithms to the link prediction prediction problem using a large set of topological features. Given a network at two different points in time, we train a learning algorithm to identify pairs of edges which appear in the newer network but not in the older network. Then, for a pair of nodes, we use the classification probability of the learning algorithm as our link prediction heuristic. Furthermore, we show that our network-specific heuristics outperform generic heuristics such as the Adamic/Adar coefficient and the Jaccard coefficient. 2 Related Work Link prediction and the application of machine learning techniques to link prediction both have significant corpuses of work behind them. Adamic and Adar used similarities between the web pages of students at MIT and Stanford to predict friendship. -
Neural Graph Representations and Their Application to Link Prediction
School of Information Sciences and Technology Department of Informatics Athens, Greece Master Thesis in Computer Science Neural Graph Representations and their Application to Link Prediction Sotiris Kotitsas Committee: Ion Androutsopoulos (Supervisor) Dimitris Pappas (Supervisor) Iordanis Koutsopoulos Haris Papageorgiou November 2020 Acknowledgements I would like to sincerely thank my supervisor Ion Androutsopoulos for the opportunity he gave me to work in this interesting eld, his support and his valuable advice. I would also like to express my heartfelt thanks to my second supervisor, Dimitris Pappas for the guidance he oered me as well as the time he invested and his positive energy. In addition, I would also like to thank Causaly for their valuable advice and the data they provided. Finally, a big thanks goes to my family for their support and especially, my friends for always being there and believing in me. ii Abstract In this thesis, we experiment with the task of Link Prediction using Network Embedding (ne) methods. ne methods map network nodes to low-dimensional feature vectors and have wide applications in network analysis and bioinformatics. We consider separately the task of Link Prediction in graphs with only one type of relationship and in graphs with more than one type of relationship. The ultimate goal is to create methods capable of making novel predictions and helping in the Biomedical domain, e.g. covid-19 related predictions. To that end, we create a biomedical dataset containing Coronavirus related information complemented by entities and relationships acquired from the umls ontology. Secondly, we note that the ne methods can be categorized to methods that utilize only the structure of the graphs and to methods that also try to exploit metadata associated with graphs, e.g. -
Link Prediction in Large-Scale Complex Networks (Application to Bibliographical Networks)
N◦ attribué par la bibliothèque | _ | _ | _ | _ | _ | _ | _ | _ | _ | _ | Université Paris Nord Doctoral Thesis Link Prediction in Large-scale Complex Networks (Application to Bibliographical Networks) Author: Manisha Pujari A thesis submitted in fulfillment of the requirements for the degree of Doctor of Philosophy in Computer Science in the research team Apprentissage Artificiel et Applications LIPN CNRS UMR-7030 Jury: Reviewer: Céline Robardet Professor INSA Lyon Bénédicte le Grand Professor Université Paris 1 Panthéon Sorbonne Examiner: Aldo Gangemi Professor SPC, Université Paris 13 Christophe Prieur Associate Professor, HDR Université Paris Diderot Director: Céline Rouveirol Professor SPC, Université Paris 13 Supervisor: Rushed Kanawati Associate Professor SPC, Université Paris 13 “ The more I learn, the more I realize how much I don’t know. ” Albert Einstein Abstract Link Prediction in Large-scale Complex Networks (Application to Bibliographical Networks) In this work, we are interested to tackle the problem of link prediction in complex net- works. In particular, we explore topological dyadic approaches for link prediction. Dif- ferent topological proximity measures have been studied in the scientific literature for finding the probability of appearance of new links in a complex network. Supervised learning methods have also been used to combine the predictions made or information provided by different topological measures. They create predictive models using various topological measures. The problem of supervised learning for link prediction is a difficult problem especially due to the presence of heavy class imbalance. In this thesis, we search different alternative approaches to improve the performance of different dyadic approaches for link prediction.