PGR Handbook Documentation Release 2019
Total Page:16
File Type:pdf, Size:1020Kb
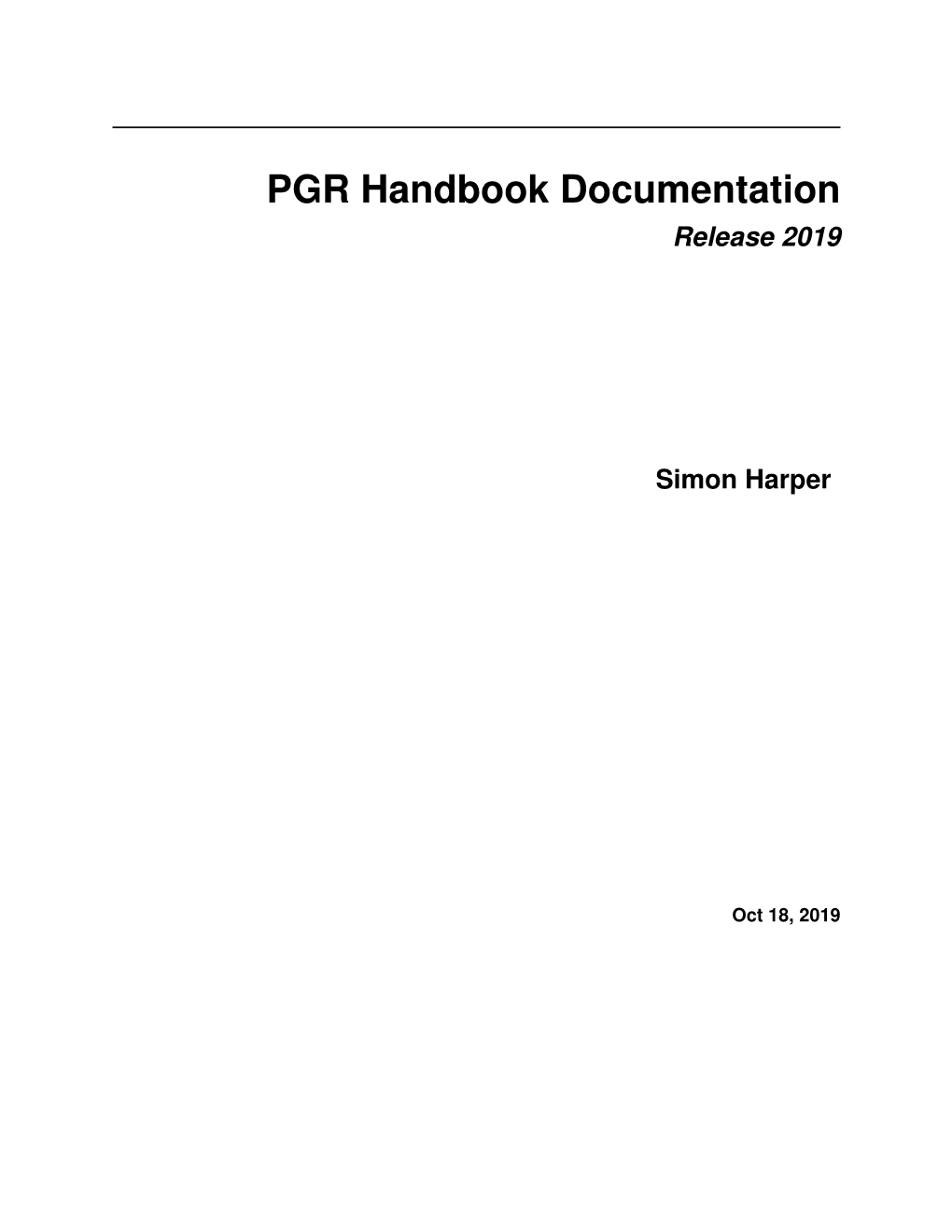
Load more
Recommended publications
-
Kaleidoquery: a Visual Query Language for Object Databases
Kaleidoquery: A Visual Query Language for Object Databases Norman Murray, Norman Paton and Carole Goble Department of Computer Science University of Manchester Oxford Road, Manchester, M13 9PL, UK E-mail: murrayn, norm, carole ¡ @cs.man.ac.uk ABSTRACT duction to one of the query environments that has been im- In this paper we describe Kaleidoquery, a visual query lan- plemented for the language, concluding with a discussion of guage for object databases with the same expressive power related work and a summary. as OQL. We will describe the design philosophy behind the filter flow nature of Kaleidoquery and present each of the LANGUAGE DESCRIPTION language’s constructs, giving examples and relating them to Graph based query languages tend to use a representation of OQL. The Kaleidoquery language is described independent the database schema as the starting point for querying the of any implementation details, but a brief description of a database. The user selects parts of the schema and constructs 3D interface currently under construction for Kaleidoquery a query from these by combining them with query operators. is presented. The queries in this implementation of the lan- A problem with graph based queries is that they depict the guage are translated into OQL and then passed to the object database schema using entity-relationship diagrams or some database O ¢ for evaluation. other notation that, while readable for a database designer, exploits an abstract symbolic notation that may at first prove KEYWORDS: Visual query language, OQL, object data- cumbersome to the casual user of a database and require bases, three-dimensional interface. -
BMC Bioinformatics Biomed Central
BMC Bioinformatics BioMed Central Introduction Open Access Proceedings of the Second International Symposium for Semantic Mining in Biomedicine Sophia Ananiadou*1 and Juliane Fluck*2 Address: 1School of Computer Science, National Centre for Text Mining, Manchester Interdisciplinary Biocentre, University of Manchester, Oxford Road, M13 9PL, Manchester, UK and 2Fraunhofer Institute SCAI, Schloss Birlinghoven, 53754 St. Augustin, Germany Email: Sophia Ananiadou* - [email protected]; Juliane Fluck* - [email protected] * Corresponding authors from Second International Symposium on Semantic Mining in Biomedicine (SMBM) Jena, Germany. 9–12 April 2006 Published: 24 November 2006 <supplement> <title> <p>Second International Symposium on Semantic Mining in Biomedicine (SMBM)</p> </title> <editor>Sophia Ananiadou, Juliane Fluck</editor> <note>Proceedings</note> </supplement> BMC Bioinformatics 2006, 7(Suppl 3):S1 doi:10.1186/1471-2105-7-S3-S1 © 2006 Ananiadou and Fluck; licensee BioMed Central Ltd. This is an Open Access article distributed under the terms of the Creative Commons Attribution License (http://creativecommons.org/licenses/by/2.0), which permits unrestricted use, distribution, and reproduction in any medium, provided the original work is properly cited. Introduction • discovery of semantic relations between entities With an overwhelming amount of biomedical knowledge recorded in texts, it is not surprising that there is so much • event discovery interest in techniques which can identify, extract, manage, integrate and exploit this knowledge, and moreover dis- The current limitations of using existing terminological cover new, hidden or unsuspected knowledge. For this and ontological resources such as the Gene Ontology, reason, in the past five years, there has been an upsurge of Swiss-Prot, Entrez Gene, UMLS, and Mesh etc. -
Alliheedi Mohammed.Pdf (7.910Mb)
Procedurally Rhetorical Verb-Centric Frame Semantics as a Knowledge Representation for Argumentation Analysis of Biochemistry Articles by Mohammed Alliheedi A thesis presented to the University of Waterloo in fulfillment of the thesis requirement for the degree of Doctor of Philosophy in Computer Science Waterloo, Ontario, Canada, 2019 c Mohammed Alliheedi 2019 Examining Committee Membership External Examiner: Vlado Keselj Professor, Faculty of Computer Science Dalhousie University Supervisor(s): Robert E. Mercer Professor, Dept. of Computer Science, The University of Western Ontario Robin Cohen Professor, School of Computer Science, University of Waterloo Internal Member: Jesse Hoey Associate Professor, School of Computer Science, University of Waterloo Internal-External Member: Randy Harris Professor, Dept. of of English Language and Literature, University of Waterloo Other Member(s): Charles Clarke Professor, School of Computer Science, University of Waterloo ii I hereby declare that I am the sole author of this thesis. This is a true copy of the thesis, including any required final revisions, as accepted by my examiners. I understand that my thesis may be made electronically available to the public. iii Abstract The central focus of this thesis is rhetorical moves in biochemistry articles. Kanoksila- patham has provided a descriptive theory of rhetorical moves that extends Swales' CARS model to the complete biochemistry article. The thesis begins the construction of a com- putational model of this descriptive theory. Attention is placed on the Methods section of the articles. We hypothesize that because authors' argumentation closely follows their experimental procedure, procedural verbs may be the guide to understanding the rhetor- ical moves. Our work proposes an extension to the normal (i.e., VerbNet) semantic roles especially tuned to this domain. -
Syntactic Analyses and Named Entity Recognition for Pubmed and Pubmed Central — Up-To-The-Minute
Syntactic analyses and named entity recognition for PubMed and PubMed Central — up-to-the-minute Kai Hakala1;2∗, Suwisa Kaewphan1;2;3∗, Tapio Salakoski1;3 and Filip Ginter1 1. Dept. of Information Technology, University of Turku, Finland 2. The University of Turku Graduate School (UTUGS), University of Turku, Finland 3. Turku Centre for Computer Science (TUCS), Finland [email protected], [email protected], [email protected], [email protected] Abstract Various community efforts, mainly in the form of shared tasks, have resulted in steady improve- Although advanced text mining methods ment in biomedical text mining methods (Kim et specifically adapted to the biomedical do- al., 2009; Segura Bedmar et al., 2013). For in- main are continuously being developed, stance the GENIA shared tasks focusing on ex- their applications on large scale have been tracting biological events, such as gene regula- scarce. One of the main reasons for this tions, have consistently gathered wide interest and is the lack of computational resources and have led to the development of several text mining workforce required for processing large tools (Miwa et al., 2012; Bjorne¨ and Salakoski, text corpora. 2013). These methods have been also succes- In this paper we present a publicly avail- fully applied on a large scale and several biomed- able resource distributing preprocessed ical text mining databases are publicly available biomedical literature including sentence (Van Landeghem et al., 2013a; Franceschini et al., splitting, tokenization, part-of-speech tag- 2013; Muller¨ et al., 2004). Although these re- ging, syntactic parses and named entity sources exist, their number does not reflect the recognition. -
Acrobat Distiller, Job 5
Information Management for Genome Level Bioinformatics - Paton, Goble Information Management for Genome Level Bioinformatics Norman Paton and Carole Goble Department of Computer Science University of Manchester Manchester, UK <norm, carole>@cs.man.ac.uk Structure of Tutorial Introduction - why it matters. Genome level data. Genomic databases. Modelling challenges. Integrating biological databases. Analysing genomic data. Summary and challenges. 213 Information Management for Genome Level Bioinformatics - Paton, Goble Information Management for Genome Level Bioinformatics Norman Paton and Carole Goble Department of Computer Science University of Manchester Manchester, UK <norm, carole>@cs.man.ac.uk Structure of Tutorial Introduction - why it matters. Genome level data. Modelling challenges. Genomic databases. Integrating biological databases. Analysing genomic data. Summary and challenges. 214 Information Management for Genome Level Bioinformatics - Paton, Goble What is the Genome? All the genetic material in the chromosomes of a particular organism. What is Genomics? The systematic application of (high throughput) molecular biology techniques to examine the whole genetic content of cells. Understand the meaning of the genomic information and how and when this information is expressed. 215 Information Management for Genome Level Bioinformatics - Paton, Goble What is Bioinformatics? “The application and development of computing and mathematics to the management, analysis and understanding of the rapidly expanding amount -
Adding Value to Scholarly Communications Through Text Mining
Adding Value to Scholarly Communications Enhancing User Experience of Scholarly Communicationthrough through Text Text Mining Mining Sophia Ananiadou UK National Centre for Text Mining • first national text mining centre in the world www.nactem.ac.uk • Remit : Provision of text mining services to support UK research • Funded by • University of Manchester, collaboration with Tokyo From Text to Knowledge Applications, users and techniques Scholarly Communication Requirements • What is needed in the repositories – Annotation and curation assistance • Creation of metadata, consistent manner – Name authorities • Merging and mapping existing resources • Prediction lists based on named entity recognition • Disambiguation – Semantic metadata creation and enhancement Provision of semantic metadata to support search • Extraction of terms and named entities (names of people, organisations, diseases, genes, etc) • Discovery of concepts allows semantic annotation and enrichment of documents – Improves information access by going beyond index terms, enabling semantic querying – Improves clustering, classification of documents • Going a step further: extracting relationships, events from text – Enables even more advanced semantic applications Semantic metadata for whom? Semantic metadata for whom? • end users – adds value to library content – allows enhanced searching functionalities – allows interaction with content, living document • automated content aggregators – access to data-driven, quality metadata derived from text • librarians – enhanced capability for semantic indexing, cross- referencing between Library collections and classification Terminology Services TerMine Identifies the most significant terms Used as metadata Suggests similar areas of interest Refines index terms for document classification Used for ontology building (Protégé TerMine plug-in) Semantic metadata: terms Term Based Applications Tag Cloud based on terms automatically extracted from the blog of BBSRC Chief Executive Professor Kell. -
Syntactic Analyses and Named Entity Recognition for Pubmed and Pubmed Central — Up-To-The-Minute
Syntactic analyses and named entity recognition for PubMed and PubMed Central — up-to-the-minute 1,2 1,2,3 1,3 1 Kai Hakala ∗, Suwisa Kaewphan ∗, Tapio Salakoski and Filip Ginter 1. Dept. of Information Technology, University of Turku, Finland 2. The University of Turku Graduate School (UTUGS), University of Turku, Finland 3. Turku Centre for Computer Science (TUCS), Finland [email protected], [email protected], [email protected], [email protected] Abstract Various community efforts, mainly in the form of shared tasks, have resulted in steady improve- Although advanced text mining methods ment in biomedical text mining methods (Kim et specifically adapted to the biomedical do- al., 2009; Segura Bedmar et al., 2013). For in- main are continuously being developed, stance the GENIA shared tasks focusing on ex- their applications on large scale have been tracting biological events, such as gene regula- scarce. One of the main reasons for this tions, have consistently gathered wide interest and is the lack of computational resources and have led to the development of several text mining workforce required for processing large tools (Miwa et al., 2012; Bjorne¨ and Salakoski, text corpora. 2013). These methods have been also succes- In this paper we present a publicly avail- fully applied on a large scale and several biomed- able resource distributing preprocessed ical text mining databases are publicly available biomedical literature including sentence (Van Landeghem et al., 2013a; Franceschini et al., splitting, tokenization, part-of-speech tag- 2013; Muller¨ et al., 2004). Although these re- ging, syntactic parses and named entity sources exist, their number does not reflect the recognition. -
Text Mining for Biomedicine an Overview: Selected Bibliography
Text Mining for Biomedicine an Overview: selected bibliography Sophia Ananiadou a & Yoshimasa Tsuruoka b University of Manchester a, University of Tokyo b National Centre for Text Mining a,b http://www.nactem.ac.uk/ (i) Overviews on Text Mining for Biomedicine Ananiadou, S. & J. McNaught (eds) (2006) Text Mining for Biology and Biomedicine, Artech House. MacMullen, W.J, and S.O. Denn, “Information Problems in Molecular Biology and Bioinformatics,” Journal of the American Society for Information Science and Technology , Vol. 56, No. 5, 2005, pp. 447--456. Lars Juhl Jensen, J. Saric and P. Bork (2006) "Literature mining for the biologist: from information retrieval to biological discovery", In Nature Reviews Genetics, Vol. 7, Feb. 2006, pp 119-129 Blaschke, C., L. Hirschman, and A. Valencia, “Information Extraction in Molecular Biology,” Briefings in Bioinformatics , Vol. 3, No. 2, 2002, pp. 1--12. Cohen, A. M., and W. R. Hersh, “A Survey of Current Work in Biomedical Text Mining,” Briefings in Bioinformatics , Vol. 6, 2005, pp. 57--71. Nédellec, C., “Machine Learning for Information Extraction in Genomics---State of the Art and Perspectives.” In Text Mining and its Applications , pp. 99--118, S. Sirmakessis (ed.), Berlin: Springer-Verlag, Studies in Fuzziness and Soft Computing 138, 2004. Rebholz-Schuhmann, D., H. Kirsch, and F. Couto, “Facts from Text—Is Text Mining Ready to Deliver? ” PLoS Biology , Vol. 3, No. 2, 2005, pp. 0188--0191, http://www.plosbiology.org, June 2005. Shatkay, H., and R. Feldman, “Mining the Biomedical Literature in the Genomic Era: An Overview,” Journal of Computational Biology , Vol. 10, No. 6, 2004, pp. -
Themes in Biomedical Natural Language Processing: Bionlp08
Edinburgh Research Explorer Themes in biomedical natural language processing: BioNLP08 Citation for published version: Demner-Fushman, D, Ananiadou, S, Cohen, KB, Pestian, J, Tsujii, J & Webber, BL 2008, 'Themes in biomedical natural language processing: BioNLP08', BMC Bioinformatics, vol. 9, no. S-11. https://doi.org/10.1186/1471-2105-9-S11-S1 Digital Object Identifier (DOI): 10.1186/1471-2105-9-S11-S1 Link: Link to publication record in Edinburgh Research Explorer Document Version: Publisher's PDF, also known as Version of record Published In: BMC Bioinformatics General rights Copyright for the publications made accessible via the Edinburgh Research Explorer is retained by the author(s) and / or other copyright owners and it is a condition of accessing these publications that users recognise and abide by the legal requirements associated with these rights. Take down policy The University of Edinburgh has made every reasonable effort to ensure that Edinburgh Research Explorer content complies with UK legislation. If you believe that the public display of this file breaches copyright please contact [email protected] providing details, and we will remove access to the work immediately and investigate your claim. Download date: 29. Sep. 2021 BMC Bioinformatics BioMed Central Research Open Access Themes in biomedical natural language processing: BioNLP08 Dina Demner-Fushman*1, Sophia Ananiadou2, K Bretonnel Cohen3, John Pestian4, Jun'ichi Tsujii5 and Bonnie Webber6 Address: 1US National Library of Medicine, 8600 Rockville Pike, Bethesda, -
Development and Analysis of NLP Pipelines in Argo
Development and Analysis of NLP Pipelines in Argo Rafal Rak, Andrew Rowley, Jacob Carter, and Sophia Ananiadou National Centre for Text Mining School of Computer Science, University of Manchester Manchester Institute of Biotechnology 131 Princess St, M1 7DN, Manchester, UK rafal.rak,andrew.rowley,jacob.carter,sophia.ananiadou @manchester.ac.uk { } Abstract in text is preceded by text segmentation, part-of- speech recognition, the recognition of named enti- Developing sophisticated NLP pipelines ties, and dependency parsing. Currently, the avail- composed of multiple processing tools ability of such atomic processing components is and components available through differ- no longer an issue; the problem lies in ensur- ent providers may pose a challenge in ing their compatibility, as combining components terms of their interoperability. The Un- coming from multiple repositories, written in dif- structured Information Management Ar- ferent programming languages, requiring different chitecture (UIMA) is an industry stan- installation procedures, and having incompatible dard whose aim is to ensure such in- input/output formats can be a source of frustration teroperability by defining common data and poses a real challenge for developers. structures and interfaces. The architec- Unstructured Information Management Archi- ture has been gaining attention from in- tecture (UIMA) (Ferrucci and Lally, 2004) is a dustry and academia alike, resulting in a framework that tackles the problem of interoper- large volume of UIMA-compliant process- ability of processing components. Originally de- ing components. In this paper, we demon- veloped by IBM, it is currently an Apache Soft- strate Argo, a Web-based workbench for ware Foundation open-source project1 that is also the development and processing of NLP registered at the Organization for the Advance- pipelines/workflows. -
2Nd Workshop on Building and Evaluating Resources for Biomedical Text Mining
2nd Workshop on Building and Evaluating Resources for Biomedical Text Mining Tuesday, 18th March 2010 Valletta, Malta Organisers: Sophia Ananiadou Kevin Cohen Dina Demner-Fushman Workshop Programme 9:15 – 9:30 Welcome 9:30 – 10:30 Invited Talk (chair: Sophia Ananiadou) Pierre Zweigenbaum, Laboratoire d'Informatique pour la Mécanique et les Sciences de l'Ingénieur (LIMSI-CNRS), France 10:30 – 11:00 Coffee break 11:00 – 12:30 Session 1 (chair: Kevin Cohen) 11:00 Spelling Correction in Clinical Notes with Emphasis on First Suggestion Accuracy Jon Patrick, Mojtaba Sabbagh, Suvir Jain and Haifeng Zheng 11:25 Automatically Building a Repository to Support Evidence Based Practice Dina Demner-Fushman, Joanna Karpinski and George Thoma 11:50 An Empirical Evaluation of Resources for the Identif cation of Diseases and Adverse Effects in Biomedical Literature Harsha Gurulingappa, Roman Klinger, Martin Hofmann-Apitius and Juliane Fluck 12:15 A Task-Oriented Extension of Chinese MeSH Concepts Hierarchy Xinkai Wang and Sophia Ananiadou 12:40 – 14:10 Lunch break 14:10 – 15:10 Invited talk (chair: Sophia Ananiadou) Simonetta Montemagni, Istituto di Linguistica Computazionale (ILC-CNR), Italy 15:10 – 16:55 Session 2 (chair: Dina Demner-Fushman) 15:10 Structuring of Status Descriptions in Hospital Patient Records Svetla Boytcheva, Ivelina Nikolova, Elena Paskaleva, Galia Angelova, Dimitar Tcharaktchiev and Nadya Dimitrova 15:35 Annotation of All Coreference in Biomedical Text: Guideline Selection and Adaptation K. Bretonnel Cohen, Arrick Lanfranchi, William -
Three Bionlp Tools Powered by a Biological Lexicon
Three BioNLP Tools Powered by a Biological Lexicon Yutaka Sasaki 1 Paul Thompson 1 John McNaught 1, 2 Sophia Ananiadou 1, 2 1 School of Computer Science, University of Manchester 2 National Centre for Text Mining MIB, 131 Princess Street, Manchester, M1 7DN, United Kingdom {Yutaka.Sasaki,Paul.Thompson,John.McNaught,Sophia.Ananiadou}@manchester.ac.uk its focus is on medical terms. Therefore some Abstract biology-specific terms, e.g., molecular biology terms, are not the main target of the lexicon. In this paper, we demonstrate three NLP In response to this, we have constructed the applications of the BioLexicon, which is a BioLexicon (Sasaki et al ., 2008), a lexical lexical resource tailored to the biology resource tailored to the biology domain. We will domain. The applications consist of a demonstrate three applications of the BioLexicon, dictionary-based POS tagger, a syntactic in order to illustrate the utility of the lexicon parser, and query processing for biomedical information retrieval. Biological within the biomedical NLP field. terminology is a major barrier to the The three applications are: accurate processing of literature within biology domain. In order to address this • BLTagger: a dictionary-based POS tagger problem, we have constructed the based on the BioLexicon BioLexicon using both manual and semi- • Enju full parser enriched by the automatic methods. We demonstrate the BioLexicon utility of the biology-oriented lexicon • Lexicon-based query processing for within three separate NLP applications. information retrieval 1 Introduction 2. Summary of the BioLexicon Processing of biomedical text can frequently be In this section, we provide a summary of the problematic, due to the huge number of technical BioLexicon (Sasaki et al ., 2008).