Desert Roughness Retrieval Using CYGNSS GNSS-R Data
Total Page:16
File Type:pdf, Size:1020Kb
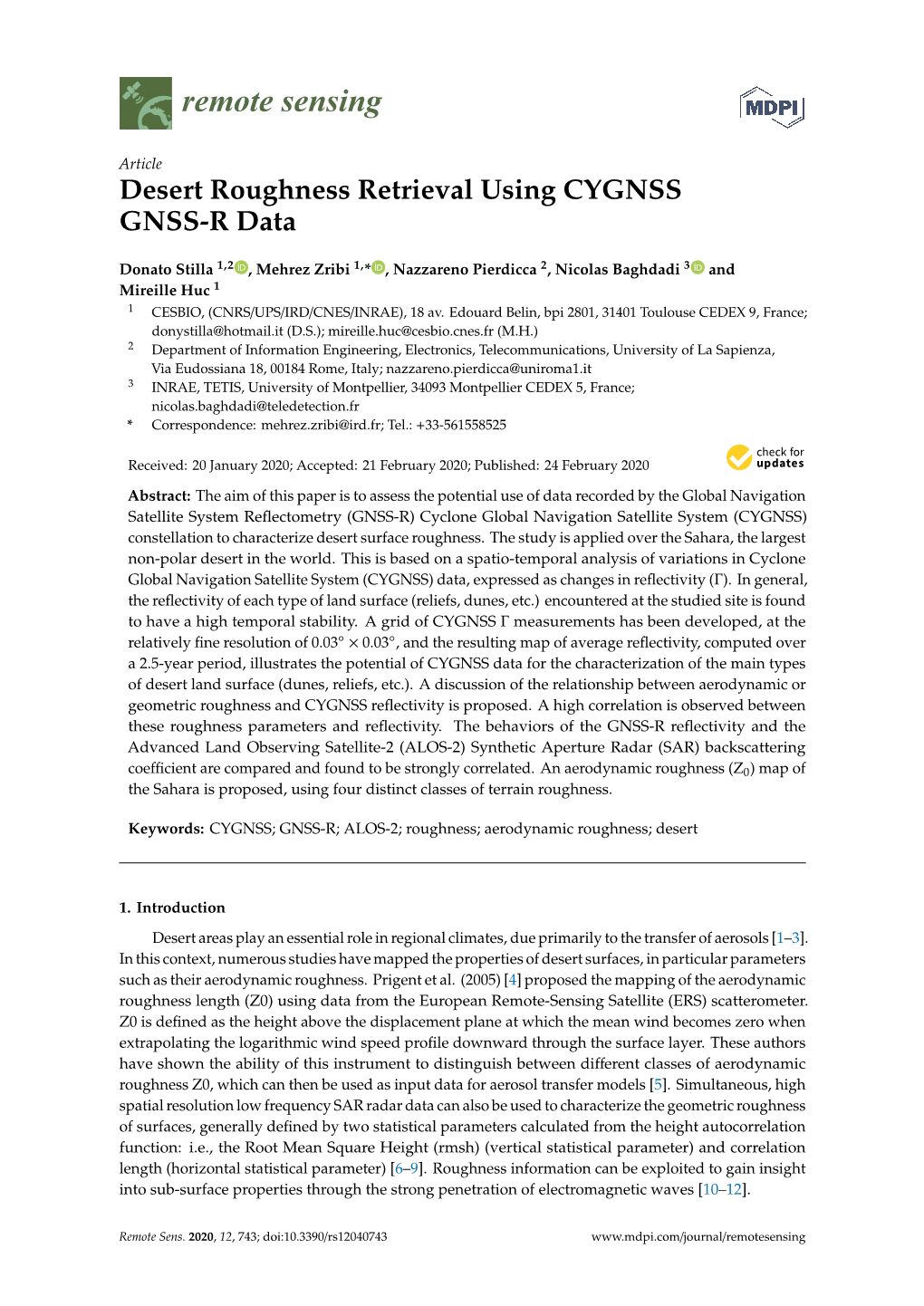
Load more
Recommended publications
-
De Smet K. 2003. Cheetah in and Around Hoggar National Park in Central Sahara (Algeria)
de Smet K. 2003. Cheetah in and around Hoggar National Park in Central Sahara (Algeria). Cat News 38(Spring):22-4. Keywords: 1DZ/Acinonyx jubatus/cheetah/Hoggar NP/National Park/North Africa/prey/Sahara/status/ territorial behaviour Abstract: According the studies conducted in the late' 80s, in 2000 and in 2003, the cheetah is largely widespread in the Hoggar Mountains (Algeria) and its surroundings. Their numbers have probably risen since 2000. The potential preys of the cheetah are abundant. However, the cheetah is often killed by the Tuaregs despite their protection status. Cheetahs are reported to move on constantly from one valley to another, but have a territorial behaviour. The absence of competitors allows cheetah to hunt its preys without facing their robbery. The situation may be good in the Tassili Mountains too. D'après les études menées à la fin des années 80, en 2000 et en 2003 le guépard est largement répandu dans les montagnes du Hoggar (Algérie) et ses environs. Leur nombre a probablement augmenté depuis 2000. Les proies potentielles du guépard sont abondantes. Cependant, malgré son statut d'espèce protégée, le guépard est souvent tué par les Touaregs. D'après les observations, les guépards se déplacent constamment d'une vallée à l'autre, mais gardent un comportement territorial. L'absence de compétiteurs permet au guépard de chasser ses proies sans avoir à faire face à leur vol. La situation doit également être bonne dans les montagnes du Tassili. Cheetah in and Around Hoggar National Park in Algeria by Koen de Smet* uring the past 80 years we encountered, and even groups up to covered 1,000 km. -
Models and Methods of Tectonic Geomorphology and the Reconstruction of Hominin Landscapes
This is a repository copy of Landscapes of human evolution : models and methods of tectonic geomorphology and the reconstruction of hominin landscapes. White Rose Research Online URL for this paper: http://eprints.whiterose.ac.uk/11178/ Version: Accepted Version Article: Bailey, G.N. orcid.org/0000-0003-2656-830X, Reynolds, Sally and King, G.C.P. (2011) Landscapes of human evolution : models and methods of tectonic geomorphology and the reconstruction of hominin landscapes. Journal of Human Evolution. pp. 257-80. ISSN 0047-2484 https://doi.org/10.1016/j.jhevol.2010.01.004 Reuse Items deposited in White Rose Research Online are protected by copyright, with all rights reserved unless indicated otherwise. They may be downloaded and/or printed for private study, or other acts as permitted by national copyright laws. The publisher or other rights holders may allow further reproduction and re-use of the full text version. This is indicated by the licence information on the White Rose Research Online record for the item. Takedown If you consider content in White Rose Research Online to be in breach of UK law, please notify us by emailing [email protected] including the URL of the record and the reason for the withdrawal request. [email protected] https://eprints.whiterose.ac.uk/ This is an author-created pdf. Cite as: In press, J Hum Evol (2010), doi:10.1016/j.jhevol.2010.01.004 Landscapes of human evolution: models and methods of tectonic geomorphology and the reconstruction of hominin landscapes Geoffrey N. Bailey 1 Sally C. Reynolds 2, 3 Geoffrey C. -
Environmental Conflicts: the Case of the Nile River Basin
Environmental Conflicts: The case of the Nile River Basin BY MAHLAKENG KHOSI MAHLAKENG 2007117787 Submitted in fulfilment of the requirements of the Master’s Degree in the Department of Political Science Faculty of Humanities at the University of the Free State. BLOEMFONTEIN JULY 2015 SUPERVISOR: MR. PA SCHOEMAN CO-SUPERVISOR: PROF. H SOLOMON i TABLE OF CONTENTS ACKNOWLEDGEMENTS ........................................................................................... V DECLARATIONS ........................................................................................................ V ABSTRACT AND KEY WORDS.…...……………………………………………………VII Key Words………………………………………………………………………………….VII Abstract…………………………………………………………………………………….VIII LIST OF ABBREVIATIONS ........................................................................................ IX CHAPTER 1: INTRODUCTION ................................................................................... 1 1.1 Orientation and background ........................................................................... 1 1.2 Problem statement and research question ..................................................... 5 1.3 Aims and objectives of the study .................................................................... 8 1.4 Research methodology .................................................................................. 8 1.5 Literature review ........................................................................................... 10 1.6 Outline of the study ..................................................................................... -
Palaeoecology of Africa*
Intimations on Quaternary palaeoecology of Africa* E.M. van Zinderen+Bakker Palynological Research Unit of the C.S.I.R. and the University of the Orange Free State at Bloemfontein 1. THE BIOGEOGRAPHIC PATTERN OF AFRICA of with of is little The biogeography Africa, as compared that Europe known and it is only in recent years that more detailed studies have been made of cer- of tain areas. Taxonomists working on groups lower plants and animals and even on flowering plants will still find many rich new areas for collecting in Africa, while the results of these taxonomic studies are the basis of any biogeo- graphic investigation. The study of the factors responsible for the present-day pattern of distribution of plants and animals has been initiated in Africa only The which directed towards fairly recently. present intimations, are mostly botanical problems, are therefore general in nature and are often hypothetical. They are only intended to indicate possible historical approaches to the study of biogeography of Africa. The flora and fauna of Africa, the continent for which some of the most an- cient radiometric dates have been assessed, must in consequence be old in origin. The priscotropical flora of the forests, savannas, mountains and deserts of Africa has been isolated from the other continents since mesozoic times and has wealth of in the flora. Botanical developed a great species especially Cape Gondwana affinities are apparent in this southern flora, while boreal influences of found in the mountainfloras from the Sa- a comparatively younger age are hara and Ethiopia in the north right down the length of the continent to the Africa. -
Quaternary Glaciation in the Mediterranean Mountains the Geological Society of London Books Editorial Committee
Quaternary Glaciation in the Mediterranean Mountains The Geological Society of London Books Editorial Committee Chief Editor Rick Law (USA) Society Books Editors Jim Griffiths (UK) Dave Hodgson (UK) Phil Leat (UK) Nick Richardson (UK) Daniela Schmidt (UK) Randell Stephenson (UK) Rob Strachan (UK) Mark Whiteman (UK) Society Books Advisors Ghulam Bhat (India) Marie-Franc¸oise Brunet (France) Anne-Christine Da Silva (Belgium) Jasper Knight (South Africa) Mario Parise (Italy) Satish-Kumar (Japan) Virginia Toy (New Zealand) Marco Vecoli (Saudi Arabia) Geological Society books refereeing procedures The Society makes every effort to ensure that the scientific and production quality of its books matches that of its journals. Since 1997, all book proposals have been refereed by specialist reviewers as well as by the Society’s Books Editorial Committee. If the referees identify weaknesses in the proposal, these must be addressed before the proposal is accepted. Once the book is accepted, the Society Book Editors ensure that the volume editors follow strict guidelines on refereeing and quality control. We insist that individual papers can only be accepted after satisfactory review by two independent referees. The questions on the review forms are similar to those for Journal of the Geological Society. The referees’ forms and comments must be available to the Society’s Book Editors on request. Although many of the books result from meetings, the editors are expected to commission papers that were not presented at the meeting to ensure that the book provides a balanced coverage of the subject. Being accepted for presentation at the meeting does not guarantee inclusion in the book. -
Seasonal Provenance Changes of Present-Day Saharan Dust
Atmos. Chem. Phys. Discuss., doi:10.5194/acp-2017-131, 2017 Manuscript under review for journal Atmos. Chem. Phys. Discussion started: 16 February 2017 c Author(s) 2017. CC-BY 3.0 License. 1 Seasonal provenance changes of present-day Saharan dust 2 collected on- and offshore Mauritania 3 4 Carmen A. Friese1, Hans van Hateren2,*, Christoph Vogt1,3, Gerhard Fischer1, Jan-Berend W. 5 Stuut1,2 6 1Marum-Center of Marine Environmental Sciences, University of Bremen, Bremen, 28359, Germany 7 2NIOZ-Royal Netherlands Institute for Sea Research, Department of Ocean Systems, and Utrecht University, 1790 8 AB, Den Burg Texel, Netherlands 9 3ZEKAM, Crystallography, Geosciences, University of Bremen, 28359, Germany 10 *Now at: Vrije Universiteit Amsterdam, Faculty of Earth Sciences, 1081 HV Amsterdam, the Netherlands 11 Correspondence to: Carmen A. Friese ([email protected]) 12 Abstract. 13 Saharan dust has a crucial influence on the earth climate system and its emission, transport, and deposition are 14 intimately related to environmental parameters. The alteration in the physical and chemical properties of Saharan 15 dust due to changes in environmental parameters is often used to reconstruct the climate of the past. However, to 16 better interpret possible climate changes the dust source regions need to be known. By analysing the mineralogical 17 composition of transported or deposited dust, potential dust source areas can be inferred. Summer dust transport 18 offshore Northwest Africa occurs in the Saharan air layer (SAL). In contrast, dust transport in continental dust 19 source areas occurs predominantly with the trade winds. Hence, the source regions and related mineralogical 20 tracers differ with season and sampling location. -
Taoudeni Basin Report
Integrated and Sustainable Management of Shared Aquifer Systems and Basins of the Sahel Region RAF/7/011 TAOUDENI BASIN 2017 INTEGRATED AND SUSTAINABLE MANAGEMENT OF SHARED AQUIFER SYSTEMS AND BASINS OF THE SAHEL REGION EDITORIAL NOTE This is not an official publication of the International Atomic Energy Agency (IAEA). The content has not undergone an official review by the IAEA. The views expressed do not necessarily reflect those of the IAEA or its Member States. The use of particular designations of countries or territories does not imply any judgement by the IAEA as to the legal status of such countries or territories, or their authorities and institutions, or of the delimitation of their boundaries. The mention of names of specific companies or products (whether or not indicated as registered) does not imply any intention to infringe proprietary rights, nor should it be construed as an endorsement or recommendation on the part of the IAEA. INTEGRATED AND SUSTAINABLE MANAGEMENT OF SHARED AQUIFER SYSTEMS AND BASINS OF THE SAHEL REGION REPORT OF THE IAEA-SUPPORTED REGIONAL TECHNICAL COOPERATION PROJECT RAF/7/011 TAOUDENI BASIN COUNTERPARTS: Mr Adnane Souffi MOULLA (Algeria) Mr Abdelwaheb SMATI (Algeria) Ms Ratoussian Aline KABORE KOMI (Burkina Faso) Mr Alphonse GALBANE (Burkina Faso) Mr Sidi KONE (Mali) Mr Aly THIAM (Mali) Mr Brahim Labatt HMEYADE (Mauritania) Mr Sidi Haiba BACAR (Mauritania) EXPERT: Mr Jean Denis TAUPIN (France) Reproduced by the IAEA Vienna, Austria, 2017 INTEGRATED AND SUSTAINABLE MANAGEMENT OF SHARED AQUIFER SYSTEMS AND BASINS OF THE SAHEL REGION INTEGRATED AND SUSTAINABLE MANAGEMENT OF SHARED AQUIFER SYSTEMS AND BASINS OF THE SAHEL REGION Table of Contents 1. -
REGIONAL GEOGRAPHY of AFRICA. Uganda Certificate of Education
REGIONAL GEOGRAPHY OF AFRICA. Uganda Certificate of Education. GEOGRAPHY Code: 273/2, Paper 2 2 hours 30 minutes PART I : THE REST OF AFRICA. INSTRUCTIONS TO CANDIDATES: This paper consists of two sections: Part I Rest of Africa. Answer two questions from part I @ question carry 25marks. Any additional question (s) answered will not be marked. Four questions are set and a candidate is required to answer only two questions. This region covers 50% of paper 273/2. 1) Download and print out a hard copy then copy this notes in a fresh book for Rest of Africa paper2. 2) If You need a copy of this work organized by the teacher for Rest of Africa. Call 0775 534057 for a book of Africa and it will be delivered. Emihen – Utec 1 SIZE, SHAPE AND POSITION. POSITION OF AFRICA. Africa is one of the largest continents of the world. It’s the second to the largest landmass combined of Eurasia i.e. Europe and Asia continents. LOCATION: Africa lies between latitudes 37.51’N just West of Cape Blanc in Tunisia to Cape Aghulhas at Latitude 34.51’S a distance of 8,000kms. Africa also lies between Cape Ras Hagun 51.50’E and Cape Verde 17.32’W. SIZE: Africa covers land area of about 30,300,300km2. THE SHAPE: Africa’s shape is unbalanced; with her northern part being bulky and wide, while the southern part being thinner and narrower in appearance. Emihen-Utec 2 The Latitude EQUATOR divides the continent into TWO HALVES, there being approximately; 3800kms between the Cape Agulhas in the south and Equator while between Tunisia and Equator in the North is 4,100kms. -
Transboundary Diagnostic Analysis of the Lake Chad Basin
Transboundary Diagnostic Analysis of the Lake Chad Basin 2018 Update Transboundary Diagnostic Analysis of the Lake Chad Basin 2018 Update 2 Transboundary Diagnostic Analysis of the Lake Chad Basin | 2018 Update Contents Executive Summary ............................................................................................................................................................................5 1 Introduction .......................................................................................................................................................................................7 1.1 Context ...............................................................................................................................................................................................8 1.1.1 The Interlinked Threats to the Lake Chad Basin ................................................................................................8 1.1.2 The Potential for Success ...............................................................................................................................................9 1.2 Objectives .......................................................................................................................................................................................10 2 Methodological Approach ..........................................................................................................................................................11 2.1 Recent Reports and Initiatives -
A Rediscovered Journey Into the Past by GOTTFRIED O. RIECK
AFRICA A rediscovered journey into the past by GOTTFRIED O. RIECK AFRICA A rediscovered journey into the past by GOTTFRIED O. RIECK Foreword Tis book is dedicated to my wife Sibylle, whom I had lef home alone for two months shortly afer our honey- moon to travel through Africa. I thought this trip was too dangerous to get a young woman into areas that had not yet been explored. Very soon I realized that my decision was perfectly right because quite ofen we were in dangerous situations, which we were only able to manage with luck and outside help. Te book was written to document a journey that would no longer be possible today. Te manuscript consists of travel and diary notes that I used to write every day addressing them to my wife to let her participate in this incredible expedition albeit indirectly. Unfortunately, those notes could not be found for a long time. But afer two decades they were rediscovered al- lowing the completion of a book about that extraordinary adventure. I must admit this trip had changed me and I returned back home mature and balanced man. With the beneft of hindsight I should say it was a daily give and take, but it was worth the efort. Gottfried O. Rieck 4 Gottfried and Sibylle The adventure begins now… 5 6 THE EXPEDITION START: 11 March 1982 Tullnerbach. Lower Austria 7:00 pm FINISH: 04 May 1982 Tullnerbach, Lower Austria 10.00 am VEHICLE: Toyota Land Cruiser Station Wagon 4200 cm3, 135 PS EQUIPMENT: 17x20 l petrol and 7x20 l water canisters, 2 tents, food for 2 months, car spare parts, tools, 1 holdall per -
March-September 2012
N°39 SAHEL AND WEST AFRICA Club MAR-SEPT 2012 Secretariat THE SAHEL AND WEST AFRICA CLUB SECRETARIAT’S NEWSLETTER IN THIS ISSUE DIRECTOR’S EDITORIAL SECURITY AND DEVELOPMENT nne de Lattre, Founder and for many years Director of the “Club du Sahel”, left us during the heart of the Dialogue on the Security-Development Nexus A summer. Her death provoked a wave of emotions within a large community of friends. To each she leaves a special heritage. Northern Mali at a glance To all and to West Africa, she leaves the Club – this particular Viewpoint by Malian Ambassador Touré space for dialogue and co-operation. Anne initiated the creation of the “Club du Sahel” in 1976 because she was convinced that the FOOD SECURITY international community could not hold West African leaders welcome an indifferent gaze to the terrible dramas the AGIR Initiative and droughts of 1973-74. At the dawn of globalisation, very little attention was paid The RPCA calls for urgent political action to this region. It was necessary to create a Food security leaders meet sustainable coalition to come to its aid. with G20 representatives Thirty-fi ve years after the creation of the Club, the Western Interview with ECOWAS Sahel is again at top of the international agenda. While during Commissioner Mr. Atouga the past decades it has made constant progress in the fi eld of Biofuels: West African farmers agriculture and in the fi ght against hunger, the Sahel has been meet with Brazilian counterparts hit by a wide range of serious international threats which found an ideal breeding ground in the fragile Sahel region. -
World Bank Document
ID Cards Listening to others The Blue Men April 1975 · ~ . Public Disclosure Authorized Neto8 about the men and 'Women oj the World Bank Group Public Disclosure Authorized plane connecting Algiers to Djanet and Tamanrasset. Listening We set out along the edge of the Tassili Plateau and the Erg d'Admer, or "Sea of Sand"; six Tuaregs will held key to guide us through the dunes and can yons to their village of Iherir, 200 communication miles away. To be' more comfortable, we dress The man recently put in charge of like them, wearing a sarwal (riding improving internal communications in pants), a gandurah (the blue robe of the Bank agrees with those who say desert dwellers), and the traditional there is a communications problem, Public Disclosure Authorized cheche (turban). Unlike other tribes but he adds that he is optimistic about of Moslem faith, it is the man among the institution's ability to improve the Tuaregs who veils his face, and itself. not the woman. This is an old custom Rene H. Springuel, in an interview that has the advantage of protecting with Bank Notes says that he consid the man's face from wind and sand ered the recent and rapid growth of on long caravan journeys. the Bank to be a major cause of the At the age of 15 or 16, men start current problem, and that it would wearing the 20-feet-long "tagilmust", take time for a larger Bank staff to concealing all but their eyes before "understand and accept" new ways of strangers. (It took us a while-and a communicating.