Analysis of Variance
Total Page:16
File Type:pdf, Size:1020Kb
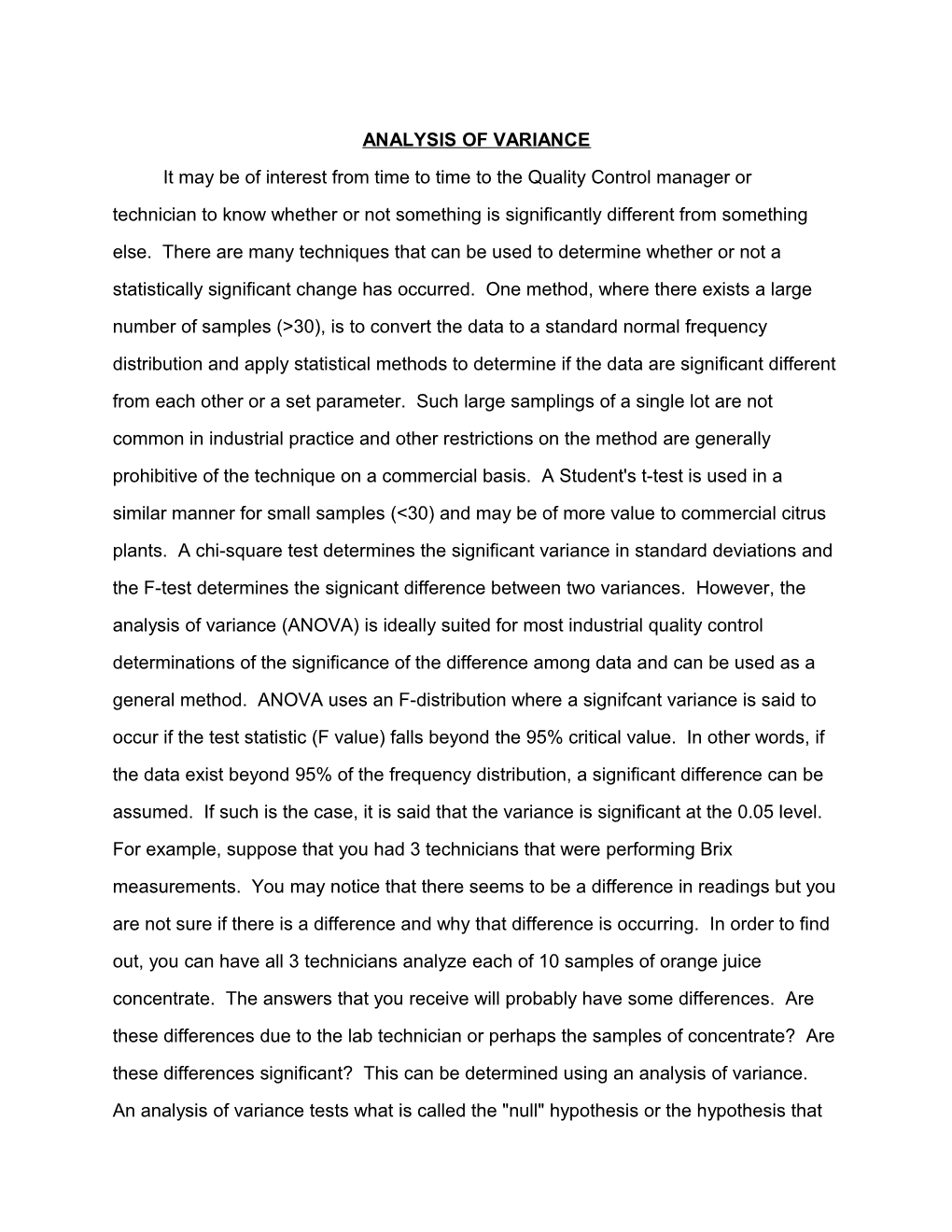
ANALYSIS OF VARIANCE It may be of interest from time to time to the Quality Control manager or technician to know whether or not something is significantly different from something else. There are many techniques that can be used to determine whether or not a statistically significant change has occurred. One method, where there exists a large number of samples (>30), is to convert the data to a standard normal frequency distribution and apply statistical methods to determine if the data are significant different from each other or a set parameter. Such large samplings of a single lot are not common in industrial practice and other restrictions on the method are generally prohibitive of the technique on a commercial basis. A Student's t-test is used in a similar manner for small samples (<30) and may be of more value to commercial citrus plants. A chi-square test determines the significant variance in standard deviations and the F-test determines the signicant difference between two variances. However, the analysis of variance (ANOVA) is ideally suited for most industrial quality control determinations of the significance of the difference among data and can be used as a general method. ANOVA uses an F-distribution where a signifcant variance is said to occur if the test statistic (F value) falls beyond the 95% critical value. In other words, if the data exist beyond 95% of the frequency distribution, a significant difference can be assumed. If such is the case, it is said that the variance is significant at the 0.05 level.
For example, suppose that you had 3 technicians that were performing Brix measurements. You may notice that there seems to be a difference in readings but you are not sure if there is a difference and why that difference is occurring. In order to find out, you can have all 3 technicians analyze each of 10 samples of orange juice concentrate. The answers that you receive will probably have some differences. Are these differences due to the lab technician or perhaps the samples of concentrate? Are these differences significant? This can be determined using an analysis of variance. An analysis of variance tests what is called the "null" hypothesis or the hypothesis that no difference exists. The limits of the "null" hypothesis are expressed in terms of probability limits or levels. If there is a certain probability that the differences can lie outside a certain range, then the difference is considered statistically significant. As mentioned previously, a common probability of 5% is used as the critical value to determine the acceptance or rejection of the "null" hypothesis. The best way to illustrate the use of an analysis of variance is by using an example. Suppose that you have three technicians that measure the Brix on 3 samples of concentrate as shown below: TECH A TECH B TECH C SAMPLE 1 61.2 61.4 60.8 SAMPLE 2 61.3 61.0 60.9 SAMPLE 3 60.9 61.2 60.9 In a one-way analysis of variance we will try to determine if there is a significant difference between the results from each technician or if one technician significantly varies from another. First, we can simplify our calculations by subtracting 60.0 from each of the numbers above to give: TECH A TECH B TECH C SAMPLE 1 1.2 1.4 0.8 SAMPLE 2 1.3 1.0 0.9
SAMPLE 3 0.9 1.2 0.9
Then the following procedure can then be used. 1.Sum each column, square the sum, add the squares and divide by the number of items in each column. For example:
(1.2+1.3+0.9)2 + (1.4+1.0+1.2)2 + (0.8+0.9+0.9)2 ─────────────────────────────────────────────── =10.43 3 2.Square each number and add. For example:
(1.2)2+(1.3)2+(0.9)2+(1.4)2+(1.0)2+(1.2)2+(0.8)2+(0.9)2+(0.9)2=10.60 3.Sum all the items, square, and divide by the total number of items. For example:
(1.2 + 1.3 + 0.9 + 1.4 + 1.0 + 1.2 + 0.8 + 0.9 + 0.9)2 ───────────────────────────────────────────────────── =10.24 9 4.Subtract the results in (3) from the results in (1). For example:
(1) - (3) = 10.43 - 10.24 = 0.19 5.Subtract the results in (3) from (2). For example: (2) - (3) = 10.60 - 10.24 = 0.36 6.Subtract the results in (4) from those in (5). For example: (5) - (4) = 0.36 - 0.19 = 0.17 With this information we can now construct an analysis of variance table. The sum of squares for the columns is found from step 4 above. The sum of squares for the residual error is found from step 6 above. The degrees of freedom (df) for the columns is found by taking the number of columns (c) and subtracting 1 or c-1. The degrees of freedom for the residual error is found from c(r-1) where "r" is the number of rows or Brix tests per technician. The mean square is found by dividing the sum of the squares by the degrees of freedom. The variance ratio can then be found by dividing the mean square of the columns by the mean square of the residual error. For example: Source Sum of Squares df Mean Square Variance Ratio columns 0.19 2 0.10 3.33 residual 0.17 6 0.03
The variance ratio can then be compared to Table 23-1 where v1 equals the degrees of freedom for the columns and v2 equals the degrees freedom of the residual. At the 0.05 probability level the variance ratio must exceed 5.14 in order for a significant difference to occur between the lab technicians. Since 3.33 is less than 5.14 we fail to reject the null hypothesis which means there is no significant difference between the lab technicians from a statistical point of view. That is not to say that the lab technicians are performing Brix measurements with sufficient accuracy. It only means that statistically they are all performing equally at the 5% level. You can expand the analysis of variance to include the determination of whether or not there is a significant difference between the concentrate samples as well as between the lab technicians. This is called a two-way ANOVA. We can use a similar example to illustrate how this is done. Suppose that we have four lab technicians that each analyzes 3 samples of orange concentrate for Brix as shown below. Tech A Tech B Tech C Tech D Sample 1 60.0 60.1 59.9 59.9 Sample 2 60.1 60.1 60.1 60.0 Sample 3 60.1 60.2 60.1 60.0 First, subtract 60.0 from each of the measurements in order to simplify the calculations. Tech A Tech B Tech C Tech D Sample 1 0 0.1 -0.1 -0.1 Sample 2 0.1 0.1 0.1 0
Sample 3 0.1 0.2 0.1 0 The following procedure can be used. 1.Sum each column, square the sum, add, and divide by the number of cases in each column. For example: ((0.2)2 + (0.4)2 + (0.1)2 + (-0.1)2 )/3 = 0.073 2.Sum each row, square the sum, add, and divide by the number of cases in each row. For example: ((-0.1)2 + (0.3)2 + (0.4)2 )/4 = 0.065 3.Square each number and add the squares. For example: 0+(0.1)2+(-0.1)2+(-0.1)2+(0.1)2+(0.1)2+(0.1)2+0+(0.1)2+(0.2)2+(0.1)2+0 = 0.120 4.Sum all the cases, square the sum, and divide by the number of cases. For example: (0+0.1-0.1-0.1+0.1+0.1+0.1+0+0.1+0.2+0.1+0)2/12 = 0.03 5.Subtract the results in 4 from those in 1. For example:
(1) - (4) = 0.073 - 0.030 = 0.043 6.Subtract the results in 4 from those in 2. For example: (2) - (4) = 0.065 - 0.030 = 0.035 7.Subtract the results in 4 from those in 3. For example: (3) - (4) = 0.120 - 0.030 = 0.090 8.Subtract the results in 5 and 6 from 7. For example: (7) - (5) - (6) = 0.090 - 0.035 - 0.043 = 0.012 With this information we can now construct an analysis of variance table. The sum of squares for the columns is found from step 5 above. The sum of squares for the rows is found from step 6 above. The sum of squares for the residual error is found from step 8 above. The degrees of freedom for the columns is found from c-1. The degrees of freedom for the rows is found from r-1. The degrees of freedom for the residual error is found from (r-1)(c-1). The mean square is found by dividing the sum of the squares by the degrees of freedom. The variance ratio can then be found for both the rows and columns by dividing the mean square of the columns and rows by the mean square of the residual error. For example: Source Sum of Squares df Mean Square Variance Ratio columns 0.043 3 0.014 7.00 rows 0.035 2 0.018 9.00 residual 0.012 6 0.002 The variance ratios can then be compared to Table 23-1 where v1 equals the degrees of freedom for the columns or rows and v2 equals the degrees freedom of the residual. At the 0.05 probability level the variance ratio must exceed 5.14 for the columns and 4.76 for the rows in order for a significant difference to occur between the lab technicians or the samples of concentrate. Since both ratios are more than 5.14 or 4.76 there is a significant difference between the lab technicians and the samples of concentrate from a statistical point of view. As you may see, this is not obvious by inspection.
Table 23-1F-distribution that can be used in the analysis of variance to test the null hypothesis at the p=0.05 level (Duncan 1974).
2 v v1=1 2 3 4 5 6 7 8 9 10 1 161 200 216 225 230 234 237 239 241 242 2 18.5 19.0 19.2 19.2 19.3 19.3 19.4 19.4 19.4 19.4 3 10.1 9.55 9.28 9.12 9.01 8.94 8.89 8.85 8.81 8.79 4 7.71 6.94 6.59 6.39 6.26 6.16 6.09 6.04 6.00 5.96 5 6.61 5.79 5.41 5.19 5.05 4.95 4.88 4.82 4.77 4.47 6 5.99 5.14 4.76 4.53 4.39 4.28 4.21 4.15 4.10 4.06 7 5.59 4.47 4.35 4.12 3.97 3.87 3.79 3.73 3.68 3.64 8 5.32 4.46 4.07 3.84 3.69 3.58 3.50 3.44 3.39 3.35
9 5.12 4.26 3.86 3.63 3.48 3.37 3.29 3.23 3.18 3.14 10 4.96 4.10 3.71 3.48 3.33 3.22 3.14 3.07 3.02 2.98 REGRESSION Regression analysis involves the fitting of data to mathematical relationships. These mathematical relationships can then be used for a variety of quality control functions. Among these is the prediction of future results. If, for example, a relationship can be found between the pulp levels before and after freshly extracted juice has passed through a seven-effect TASTE evaporator then this relationship can be used to predict the pulp level change in the future under similar conditions. Another common used of regression analysis is the conversion of data into a form that can easily be placed into a programmable device such as a calculator, computer, bar code system, or automated processing controls. This has been done with sucrose Brix/density tables and Brix corrections used extensively in the text. Regression analysis simply means fitting a line or curve to data points plotted on a graph. This can be done by simply drawing a line or curve by eye or seeking a somewhat simple mathematical relationship that may be somewhat obvious by the data. However, a statistical procedure exists that enables the calculation of such relationships as well as an objective way to determine the validity of such relationships. This method is call the method of least squares. Least Squares Analysis. Least squares' analysis involves the use of differential calculus in determining the equation that best fits a set of data. However, once the relationships have been established, computers can be easily programmed to handle the bulk of the calculations. The simplest example is determining the best equation for a straight line that fits the data or y = a + bx. Here "b" is the slope of the line and "a" is the y-intercept. In order to find the best values of "a" and "b" that will fit the data we must sum the squares of the differences between the actual "y" values and the predicted "yp" values.
yp = a + bx (23-4)
2 2 = Σ (y - yp) = Σ(y - a - bx) D (23-5) The best "a" and "b" values are those when this sum of the squares is at a minimum. In order to find the minimum point on this equation we can try to find the point where the slope is zero. This we can do by partially differentiating equation 23-5 with respect to each coefficient, and setting the result equal to zero.
δD/δa = Σ 2(y - a - bx)(-1) = 0 (23-6) δD/δb = Σ 2(y - a - bx)(-x) = 0 (23-7) Rearanging the above equations gives us: an + bΣx = Σy (23-8)
aΣx + bΣx2 = Σxy (23-9) This gives us two equations to find the two unknowns "a" and "b". Here "n" is the number of (x,y) data pairs used in the analysis. Using equations 23-8 and 23-9 to solve for "a" and "b" we obtain the following.
Σx2Σy - ΣxΣxy a = ──────────────── (23-10) nΣx2 - (Σx)2
nΣxy - (ΣxΣy) b = ───────────────── (23-11) nΣx2 - (Σx)2
Each of the above are independent equations. The equation for "a" can be simplified by first calculating "b" and then use the following dependent equation.
a = yavg - bxavg (23-12) Thus, using these two equations, we can calculate the best values for the constants "a" and "b" by performing the above summations using the sample data points "x" and "y" and the number of data pairs "n". For example, suppose we were interested in finding a linear equation that would best describe the change of pulp level in freshly extracted orange juice before and after evaporation to 65oBrix. We would first obtain the following data. SAMPLE % PULP BEFORE EVAP (x) % PULP AFTER EVAP(y) 1 16.5 10.6 2 15.9 8.8 3 16.9 10.5 4 17.4 11.4 5 18.6 11.7 Using equations 23-10 and 23-11 we obtain the following parameters and the resulting best "a" and "b" values.
Σx = 85.3 a = -6.21
Σy = 53.0
Σx2 = 1459.4 b = 0.985
Σxy = 908.3
We can then make a statistical prediction of pulp levels before and after evaporation using the resulting equation.
% pulpafter = 0.985(% pulpbefore) - 6.21 (23-13)
Using this equation we can calculate predicted values and compare them to the actual values. MEASURED % PULP AFTER EVAP CALCULATED % PULP AFTER EVAP 10.6 10.0 8.8 9.5 10.5 10.4 11.4 10.9 11.7 12.1
This is a simple way to check the accuracy of the linear least squares best fit of the data. However, the "goodness" of fit can be calculated mathematically with more objective results using the correlation coefficient R2. The closer the correlation coefficient is to 1.00, the better the data fits the regression equation. If the R2 equals exactly 1.00, the curve fits the data exactly and exact predictions can be made. For a linear equation the R2 value can be calculated from:
n(Σxy) - (Σx)(Σy) R2 = ────────────────────────────── (23-14) n(Σx2) - (Σx)2 n(Σy2) - (Σy)2
In the example above the R2 value becomes 0.646 or 64.6% of the "y" values (% pulp after evaporation here) that can be accounted for using this least squares equation. It is important to keep in mind that "noisy" variations will give a lower correlation coefficient than smooth or stretched deviations from the regression line. Actual and calculated values should be checked to verify the correlation. Also, the regression equation is usually valid only within the range of the data. The linear least squares' equation determined above may not fit the data as closely as you would like. Since the above results represent the best linear fit to the data, the only way to obtain a better fit is to use another equation, a non-linear equation. Using the same principles above, and substitution, the method of least squares can be applied to a wide variety of equations. The least squares' analysis can be programmed into computers for easy use. The reader is referred to Kolbe (1984) who has not only provided the equations needed to fit data to up to 19 different equations but has provided computer programs in a variety of program languages that can be used to determine regression relationships automatically.